The Dilemma of Modern Data Stacks Part 2
The rapid growth in data’s volume, variety, and velocity has exposed the inefficiency of traditional processes in preserving the quality of contemporary data. These legacy systems, once effective in less complex data scenarios, now struggle to scale and adapt to the intricacies and magnitude of the current data landscape.
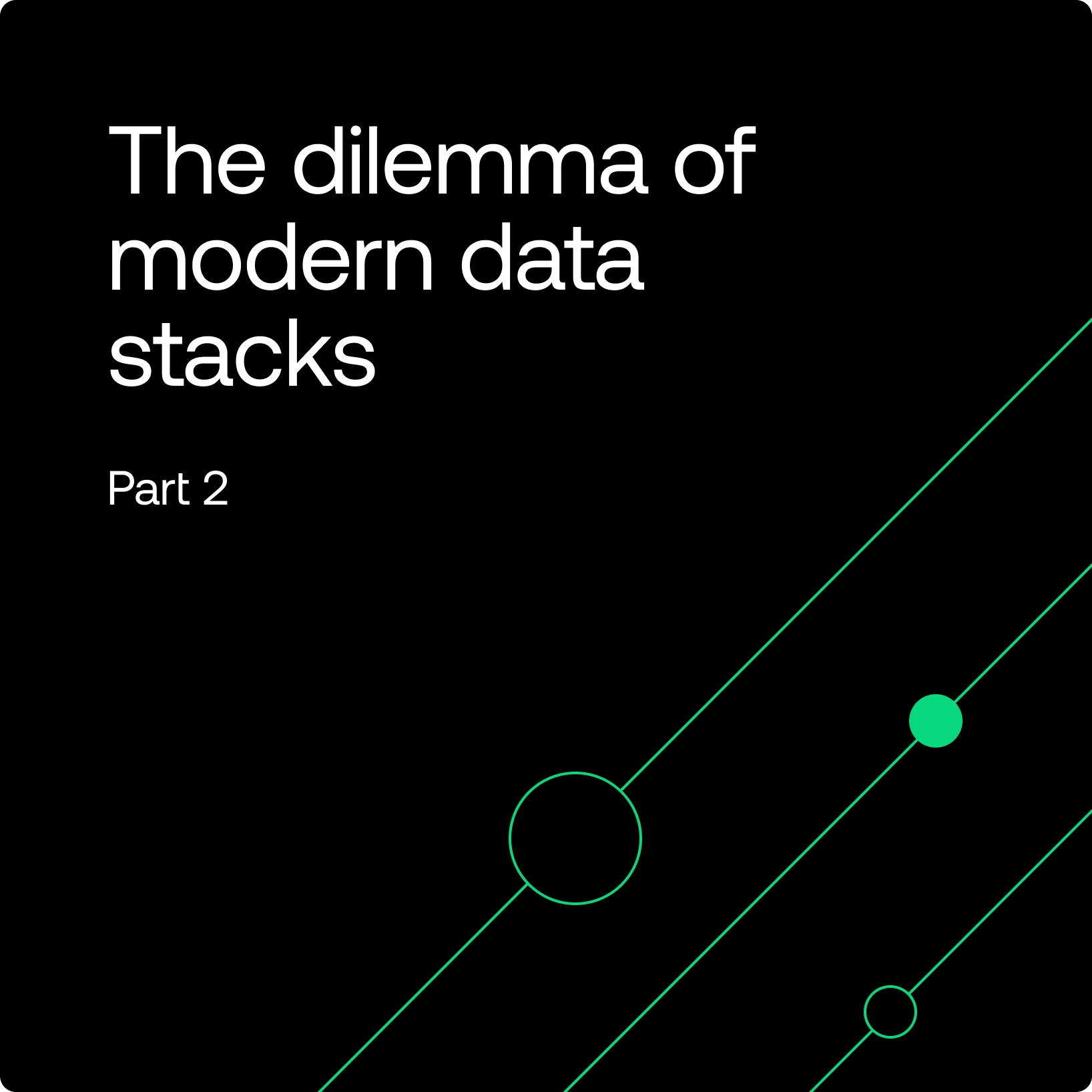
August 1, 2023
How to Improve Data Quality with the Not So Modern Data Quality Techniques?
In part 1 of this article, we discussed the importance of data quality in today’s data landscape and looked at the shortcomings of traditional approaches in great detail. In this blog, we will discuss how data quality tools have evolved over time to best fit today’s modern data architectures and the use cases they can achieve.
Evolution of Data and The Inefficiency of Legacy Processes in Maintaining Quality of Modern Data
The evolution of data, characterized by its exponential volume, variety, and velocity growth, has rendered legacy processes inefficient in maintaining modern data quality. While these processes may have been suitable for simpler data environments, they need help to meet the scalability requirements imposed by the complexity and scale of today’s data landscape.
As data architectures have evolved, traditional data warehouse and lake house architectures have given way to more advanced approaches, such as data fabric and mesh architectures. These new architectures are designed to handle distributed data sources, facilitate seamless data integration, and enable efficient data governance and quality management.
Legacy quality management processes need more flexibility and agility to seamlessly integrate and manage data from diverse sources across different locations.
This limitation leads to data inconsistencies, delays in data availability, and challenges in ensuring data accuracy and reliability.
Moreover, manual data validation, cleansing, and integration processes are time-consuming, error-prone, and difficult to scale.
These inefficiencies of traditional processes pose significant challenges for modern data use cases such as artificial intelligence, machine learning, and real-time analytics, which rely on high-quality, accurate, and timely data for optimal performance and decision-making.
Embracing modern data quality practices and technologies has become essential to unleashing the full potential of AI, ML, and real-time analytics in leveraging data for actionable insights and competitive advantage.
How to Improve Data Quality for Modern Data Ecosystems?
Here are several approaches that can help organizations enhance data quality in their modern data ecosystem:
- Automated Data Observability
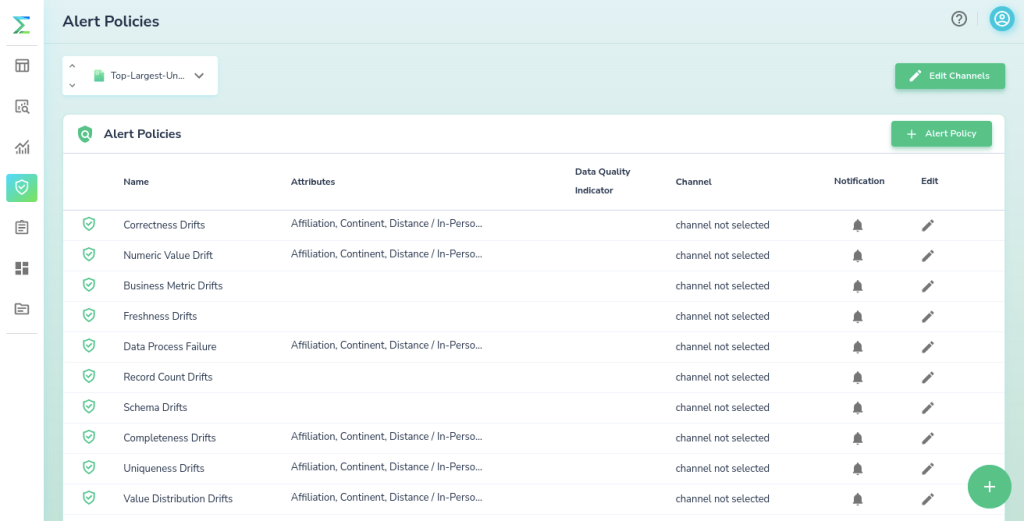
Telmai enables users to define alert policies on their datasets
Improving data quality in the modern data ecosystem requires implementing automated data observability practices. To achieve this, organizations can take the following steps:
- Leverage automated machine learning-driven anomaly detection techniques. This enables the timely identification of data abnormalities and deviations from expected patterns, allowing for proactive resolution of data quality issues, without any prior knowledge of data.
- Implement real-time, end-to-end pipeline monitoring that oversees all data types flowing through the system. This comprehensive oversight ensures consistent data quality throughout the entire data lifecycle.
- Automate bad data handling by incorporating robust data validation and cleansing processes. This helps mitigate the impact of low-quality data on downstream analyses and decision-making.
- Conduct quality checks for both the data itself and its metadata. Data checks ensure that data is complete, accurate, consistent, and relevant and metadata quality checks ensure data reliability and enable effective data lineage tracking.
- Establish and monitor data quality metrics to ensure adherence to defined quality standards. This provides a benchmark for data quality and enables organizations to track improvements over time.
- Building Data Contracts for Cross-Team Collaboration
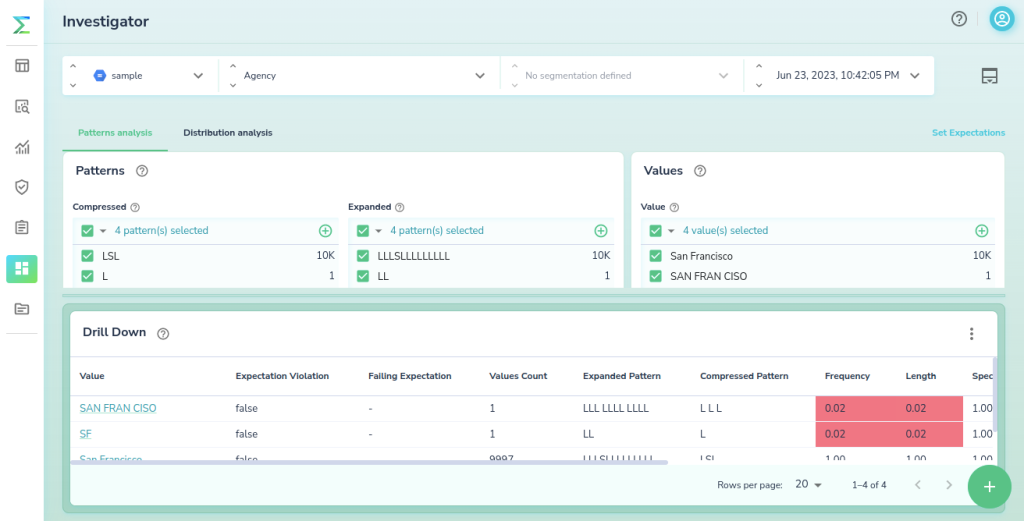
Telmai enables team members to investigate data quality issues
Data contracts are crucial in improving data quality in modern data ecosystems. They establish clear guidelines and expectations for cross-team collaboration. Moreover, they serve as agreements that define how data should be structured, governed, and managed, ensuring consistency and reliability throughout the data lifecycle. Establishing data contracts enables:
- Preventing unexpected schema changes by defining and documenting the expected structure and format of data, teams can ensure consistent data representation. Thereby reducing the risk of data inconsistencies and compatibility issues.
- Specification of data domain rules that outline the standards and guidelines for data within specific domains. Adhering to these rules enables data teams to maintain high-quality data across different systems and processes.
- Setting and enforcing Service Level Agreements (SLA) that define expectations regarding data quality, availability, and timeliness. A clear definition of SLAs allows teams to align their efforts and ensure accountability for meeting data quality requirements.
- Specifying data governance principles, including clear guidelines for data access, ownership, privacy, and security. A well-defined governance framework helps organizations ensure compliance, maintain data trustworthiness, and mitigate data handling risks.
- Maintaining a Reliable Data Catalog
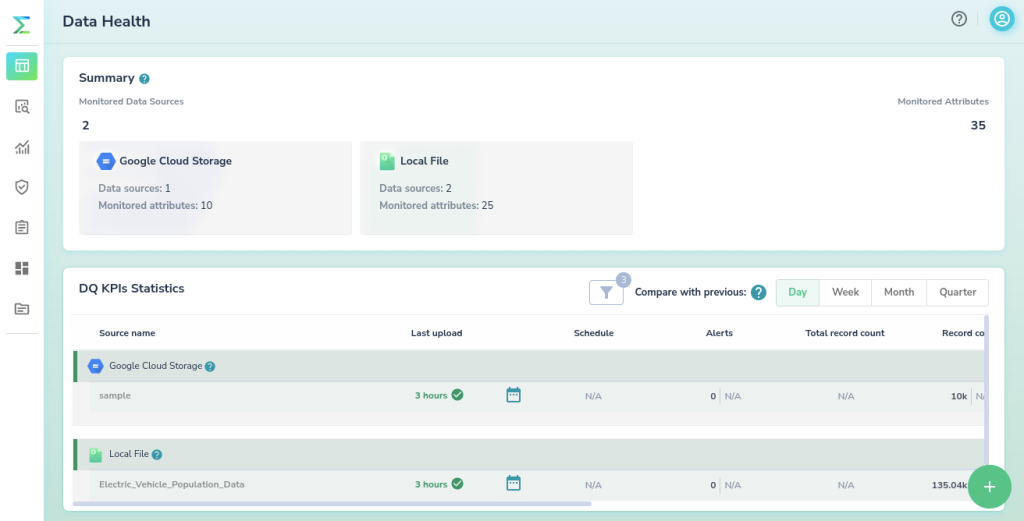
Telmai integrates with leading data catalogs to keep the the health information assets up to date
A data catalog serves as a centralized repository that provides comprehensive information about available data assets, their structure, semantics, and lineage. A well-maintained data catalog enables organizations to enhance their data quality by:
- Ensuring data discoverability that enables easy data accessibility and understandability. It provides teams with a clear overview of data sources, their formats, and relevant metadata, ensuring efficient and accurate data exploration.
For example, Google’s Earth Engine is a vast catalog of satellite imagery and geospatial datasets, empowering researchers to rapidly and accurately discover changes, map trends, and quantify differences on Earth’s surface from anywhere in the world.
- Facilitating data understanding and validation by enabling data consumers to access detailed information about the data’s origin, quality, and transformations applied to it. This enables users to assess data suitability, identify potential issues, and ensure data quality meets their specific requirements.
- Promoting data governance by enforcing standardized data management practices. It enables the documentation of data ownership, stewardship responsibilities, and data governance policies. This ensures that data is managed consistently across the organization, promoting data quality, compliance, and accountability.
- Supporting data lineage tracking by capturing the end-to-end data journey from source to consumption. This lineage information helps understand data transformations, identify potential bottlenecks, and ensure data quality and integrity throughout the data flow.
- Strict Adherence to Data Compliance & Regulation
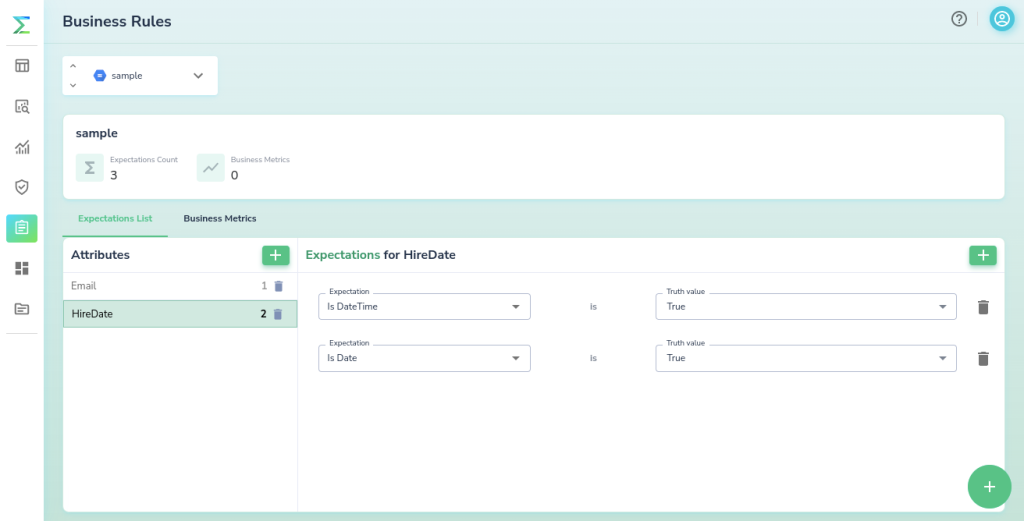
Telmai enables users to define strict business rules to capture inaccuracies
- Improving data quality in modern data ecosystems necessitates meeting the regulatory requirements of various governing bodies. Compliance with these data regulations is crucial for protecting individuals’ privacy rights and ensuring responsible data handling practices.
- Key regulations include GDPR (General Data Protection Regulation) for Europe, CCPA (California Consumer Privacy Act) for California, and other regional and state-level data regulation laws in the United States.
- Data regulation laws typically require organizations to obtain explicit consent for data collection, storage, and processing activities. They mandate transparency in data handling practices, necessitating clear privacy policies and disclosures. Data regulation laws often grant individuals the right to access, modify, or remove their personal data upon request.
- Strict data compliance and regulation adherence ensure legal and ethical data collection and processing. This fosters trust among organizational stakeholders, enhances data privacy, and mitigates non-compliance risks. A standardized approach to data handling promotes data quality and integrity and establishes a solid foundation for responsible data management in modern data ecosystems.
Achieve End-to-End Data Quality with the Leading Data Observability Tool: Telmai
In today’s data-driven world, where information is gold, data becomes increasingly valuable to organizations, making the cost of poor data quality even more significant. As organizations continue to seek effective solutions that can mitigate these financial implications and drive business success, embracing a robust data observability platform emerges as a game-changing strategy to ensure data quality in the dynamic data landscape. Enter Telmai.
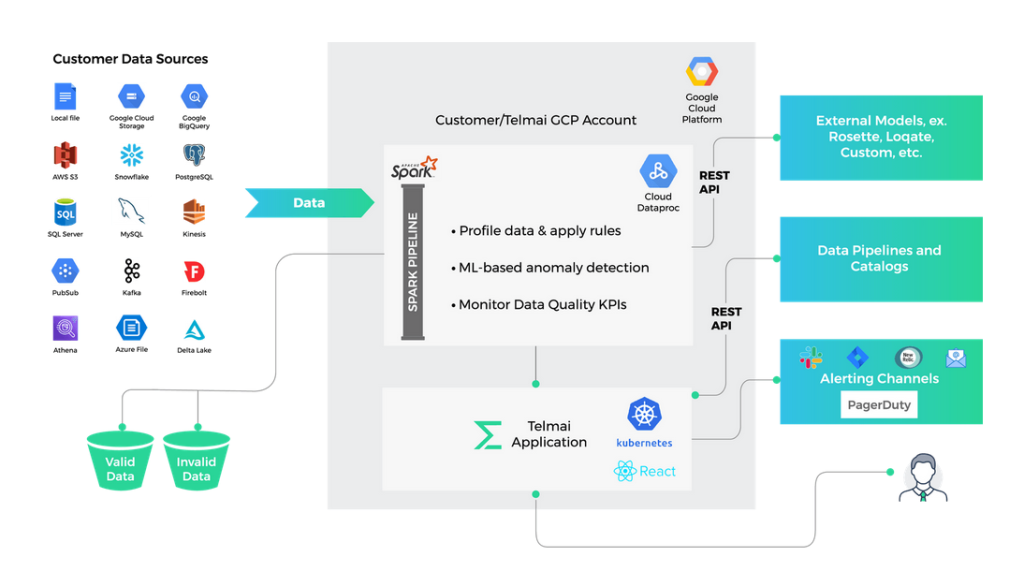
Telmai is the ultimate no-code, low-code, centralized observability solution for data teams to detect and investigate anomalies in real-time proactively. It is equipped with cutting-edge features that enable data teams to ensure high data quality in their modern data ecosystem, including multi-tenant data observability, data profiling, ML-based anomaly detection, and data quality KPIs monitoring.
Embark on your data observability journey today to unlock the full potential of your valuable data assets for data-driven excellence. Request a demo today and experience the game-changing data quality management capabilities of Telmai firsthand.
- On this page
See what’s possible with Telmai
Request a demo to see the full power of Telmai’s data observability tool for yourself.
Articles
See all articles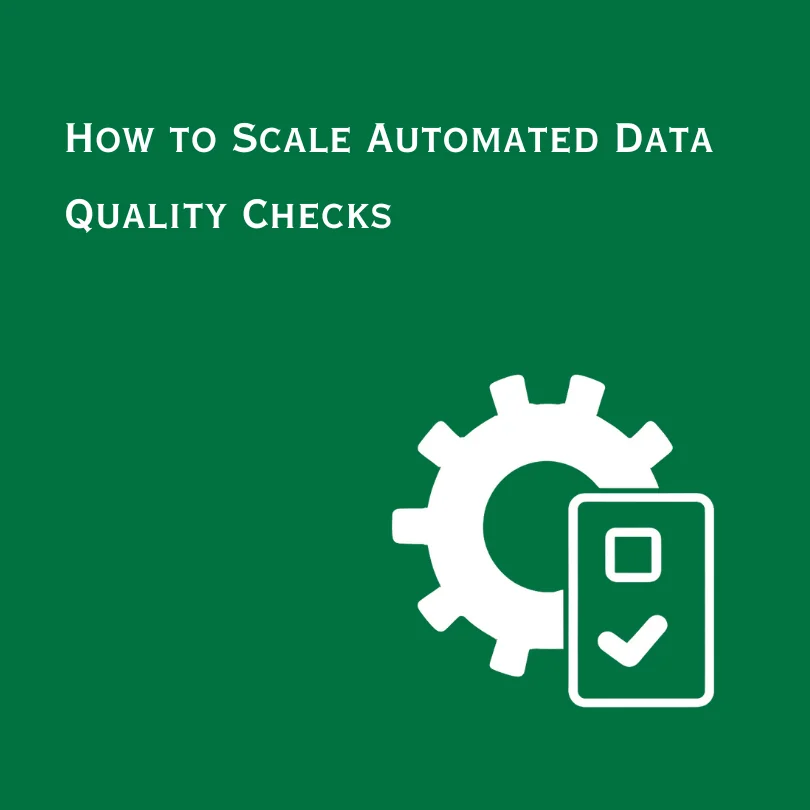
How to Scale Automated Data Quality Checks
May 01, 2024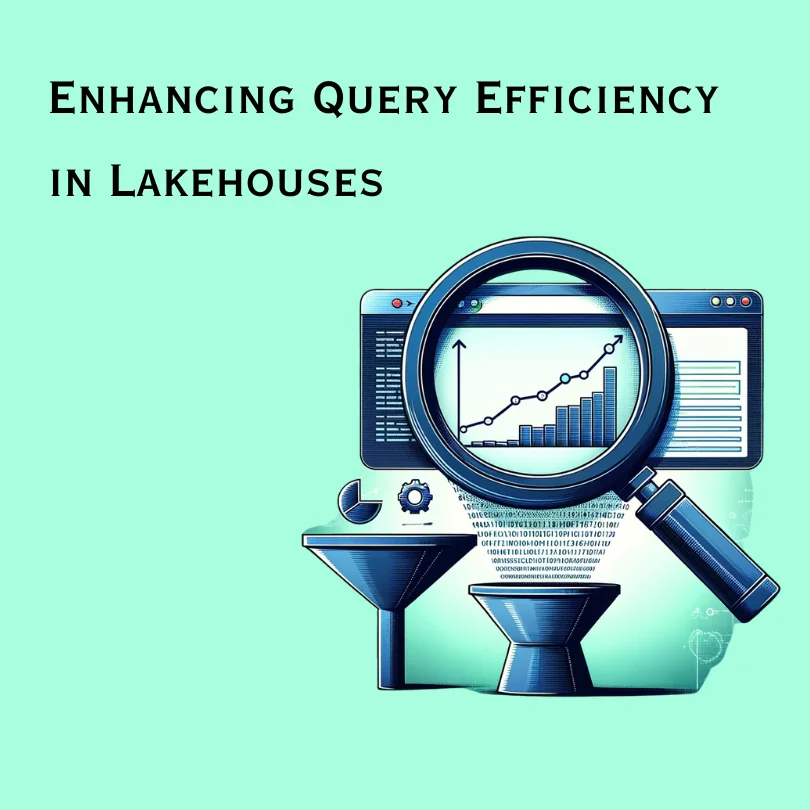