How to Improve Data Quality in a Modern Data Ecosystem
Data quality, akin to the crucial yet often ignored fine print in a contract, is pivotal and can’t be overlooked, especially when creating desirable products. As companies delve further into areas like AI, machine learning, and real-time analytics, ensuring data isn’t the weak link becomes even more critical.
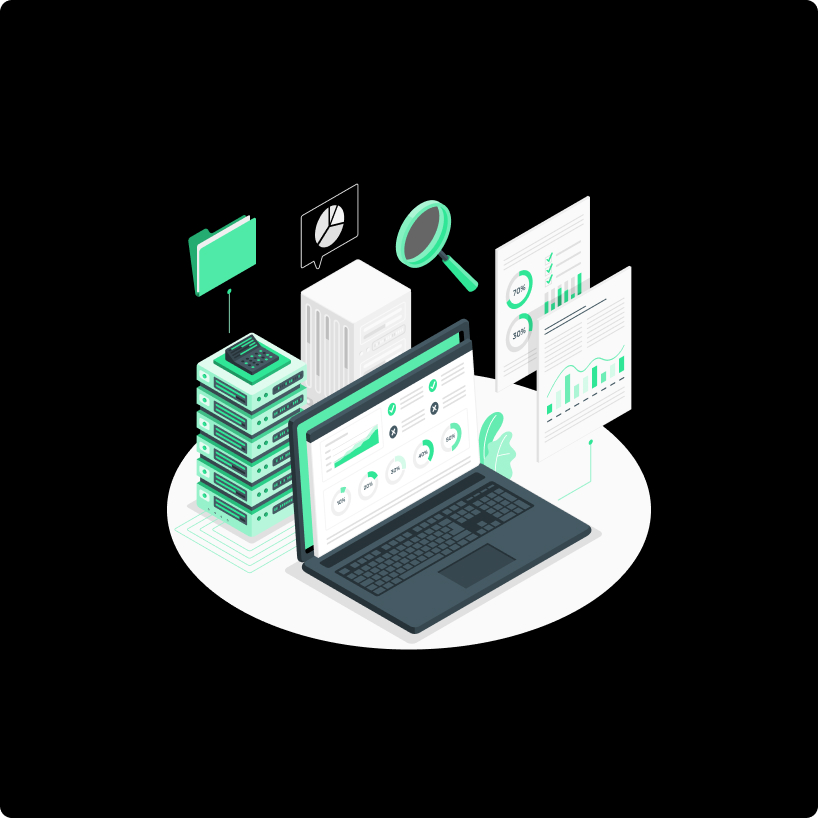
July 20, 2023
Data quality is like the fine print in a contract, easily overlooked but absolutely pivotal. It’s often something nobody reads until, well, they have to. But if you’re in the business of making things people want, then you’re also in the business of guaranteeing your data isn’t the weak link.
And now with companies venturing deeper into realms like AI, machine learning, and real-time analytics, the stakes are higher than ever.
Yet, there’s a chasm. A recent Gartner survey spotlighted the jarring reality: less than half of data and analytics teams effectively provide value to their organizations. It’s the classic tale: the giants of industry are playing 4D chess while some are, let’s say, still setting up the board. So, how do you make the right moves to ensure data quality? And more importantly, can you do so without flipping the board in frustration?
Below, we’ll discuss the importance of data quality, the shortcomings of traditional approaches, and how data quality tools and processes have evolved to fit today’s data landscape.
First, What is Data Quality?
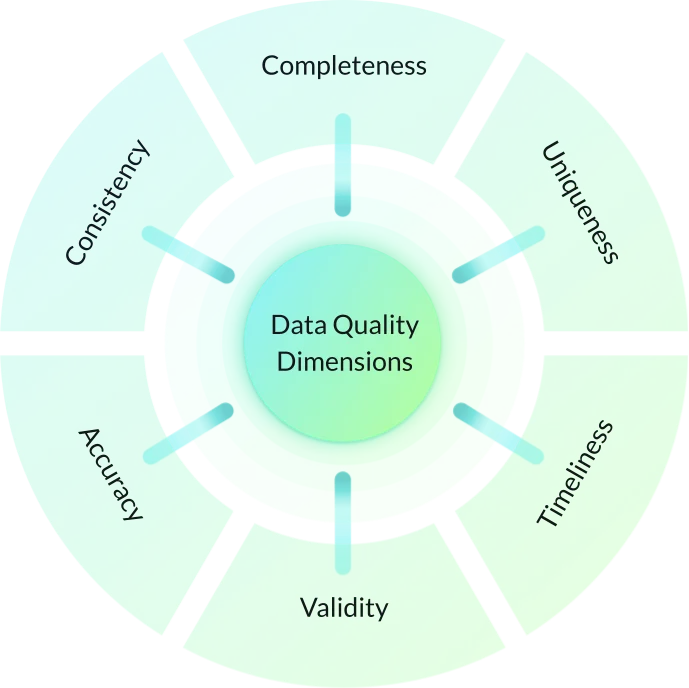
Data quality is a measure of the fitness of data for its intended purpose in an organization.
To build reliable pipelines that ensure high-quality data for effective analysis, decision-making, and other business purposes, data must meet the following six characteristics:
To build reliable data pipelines to ensure high-quality data for effective analysis, decision-making, and other business purposes, data teams must provide the following 6 data quality characteristics:
- Accuracy: Data should be free from errors or inaccuracies, with duplicate data and/or errors identified and merged or removed, ensuring it reflects true values and facts.
- Completeness: Data should be comprehensive and include all relevant information without any missing values or gaps, providing a holistic view.
- Validity: Data should adhere to defined rules, constraints, and standards, meeting the specified criteria for its intended use, e.g. through data imputation to manage missing values.
- Consistency: Data should be uniform and coherent across different sources, systems, or timelines, maintaining standardized and consistent formats, definitions, and structures.
- Timeliness: Data should be up-to-date and reflect the most recent information available, allowing users to make informed decisions based on current insights.
- Uniqueness: Data records should be distinct and non-duplicated, ensuring data integrity and preventing redundancy.
Shortcomings of Traditional Data Quality Processes
Legacy data quality processes are ill-equipped for the intricacies of modern data. Originally fit for simpler data ecosystems, they lack the needed scalability, aren’t agile enough, and have difficulty integrating and managing data from diverse sources across different locations. This can lead to inconsistencies, delays, and concerns about accuracy.
Let’s get into the details of some of these risks:
- Errors with Manual Validation: Traditional ecosystems rely heavily on manual data quality and validation checks, making them time-consuming, error-prone, and less scalable.
- Limited Data Observability: Without automated data monitoring, continuous visibility into data pipelines and processes is lacking, affecting accuracy and reliability.
- Centralized Data Ownership: Legacy systems typically use centralized data storage, which can be unsuitable for organizations with diverse data systems. This siloed approach hinders data integration, sharing, collaboration, and effective quality/governance management by domain teams.
- Undefined Data Governance: Without clear governance principles you’ll have inconsistent practices, varying standards, and accountability issues.
- Reactive Approach to Data Quality: Legacy systems tend to address data quality issues reactively, neglecting proactive measures like data profiling, cleansing, and upstream quality control, leading to persistent quality challenges, increased correction costs, and data process disruptions.
- Limited Metadata and Data Lineage: The lack of robust metadata management and data lineage tracking impairs data understanding, impact analysis of errors, and quality control efforts.
- No Data Catalogs: The absence of centralized, searchable data repositories in many traditional ecosystems limits transparency, discoverability, and collaboration, affecting tracking and management of data quality issues.
How Do You Improve Data Quality Today?
As traditional data warehouse and lake house architectures have evolved to more advanced architectures such as data fabric and data mesh, so too must your approach to data quality evolve.
Three ways to improve data quality within these modern architectures are by leveraging data observability, building data contracts for cross-team collaboration, and maintaining a reliable data catalog.
Automate Data Observability
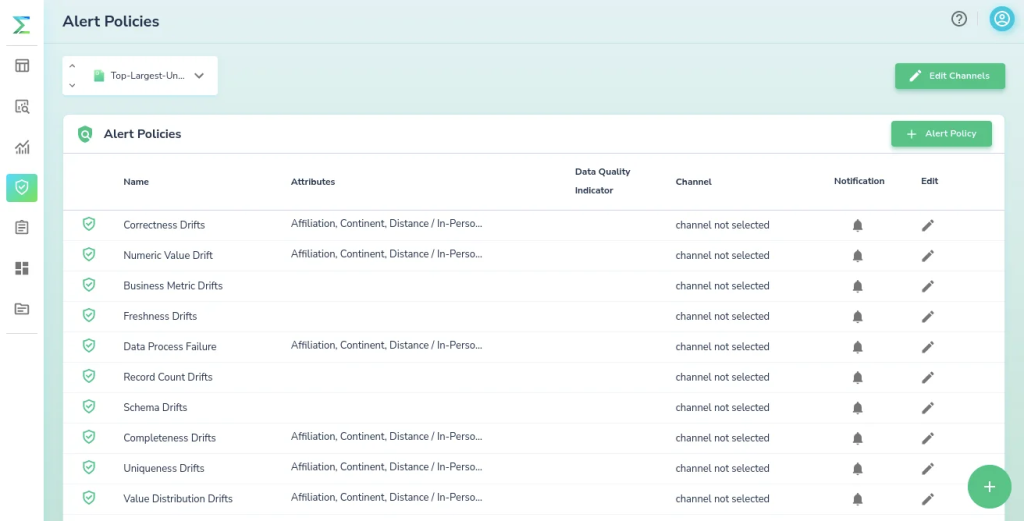
Implementing automated data observability practices is essential to improving data quality.
With machine learning-driven anomaly detection techniques and real-time end-to-end pipeline monitoring, a data observability platform like Telmai enables the timely identification of data abnormalities and ensures consistent data quality throughout the entire data lifecycle.
Quality checks, for both data and its metadata, and bad data handling can all be automated, enabling effective data lineage tracking and mitigating the impact of low-quality data on downstream analyses and decision-making.
With so many of these processes handled automatically, organizations can focus instead on establishing and monitoring data quality metrics, and track improvements to those metrics over time.
Build Data Contracts for Cross-Team Collaboration
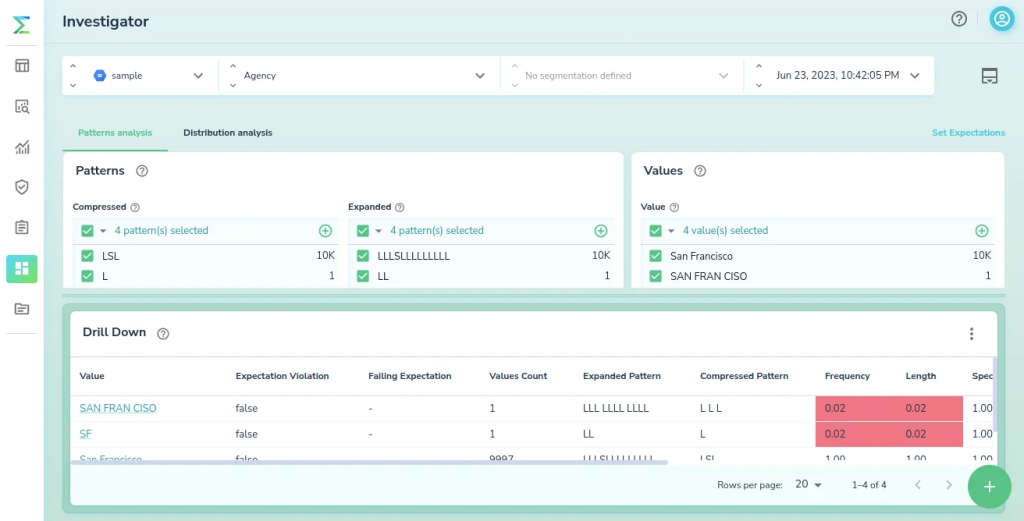
Data contracts, which establish clear guidelines and expectations for cross-team collaboration, are crucial in improving data quality in modern data ecosystems.
Specifically, data contracts serve as agreements that define how data should be structured, governed, and managed, ensuring consistency and reliability throughout the data lifecycle.
Establishing data contracts can help organizations in the following ways:
- Prevent unexpected schema changes by defining and documenting the expected structure and format of data, reducing inconsistencies and compatibility issues
- Specify data domain rules that outline standards and guidelines for data, maintaining quality across different systems and processes
- Ensure accountability, with the setting and enforcement of Service Level Agreements (SLAs) that define expectations regarding data quality, availability, and timeliness
- Specify data governance principles (e.g. guidelines for data access, ownership, privacy, security), ensuring compliance and mitigating data handling risks
In short, data contracts allow teams to align their efforts, ensure accountability for meeting data quality requirements, and contribute to the overall quality of organizational data.
Maintain a Reliable Data Catalog
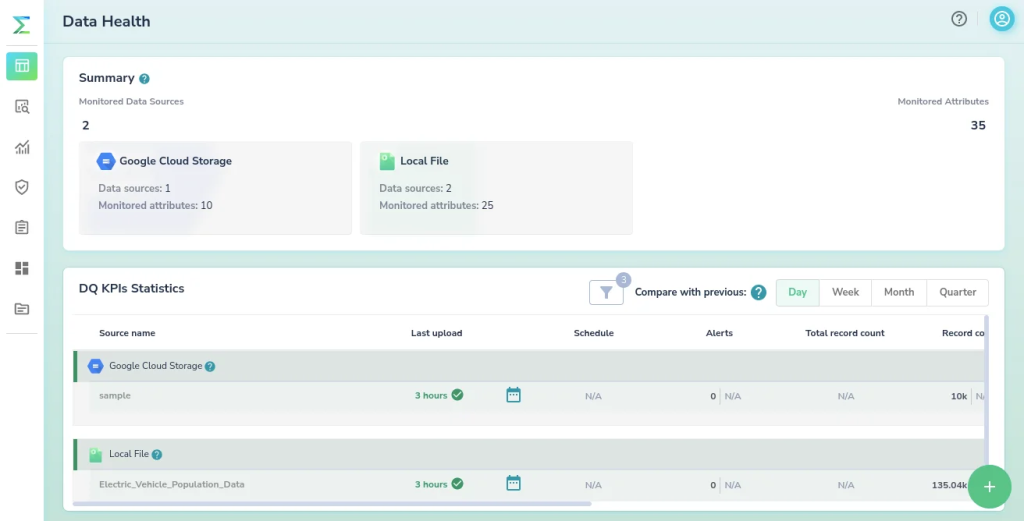
A data catalog serves as a centralized repository that provides comprehensive information about available data assets, their structure, semantics, and lineage.
Armed with information about data’s origin, quality, and transformations applied to it, users can assess its suitability, identify potential issues, and ensure it meets their specific requirements. Meanwhile, data lineage tracking (from source to consumption) helps providers and consumers alike ensure data quality and identify potential bottlenecks.
Data catalogs also promote data governance by enforcing standardized data management practices. They enable the documentation of data ownership, stewardship responsibilities, and other policies, ensuring that data is managed consistently across the organization.
Achieve End-to-End Data Quality with Telmai
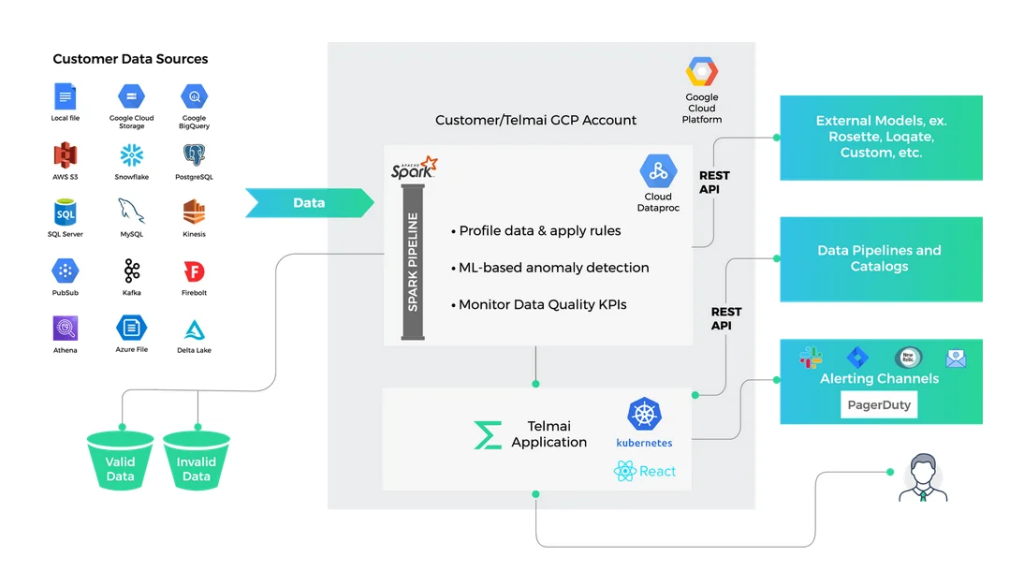
Telmai is the ultimate no-code/low-code data observability solution for data teams to proactively detect and investigate anomalies in real time. The platform is packed with cutting-edge features like multi-tenant data observability, data profiling, ML-based anomaly detection, and data quality KPI monitoring.
In other words, it’s the perfect tool for data teams looking to ensure high quality in their modern data ecosystem, mitigate the financial implications of bad data, and drive business success. Embark on your data observability journey today to unlock the full potential of your valuable information assets and achieve data-driven excellence.
Request a demo today to experience the game-changing capabilities of Telmai firsthand.
- On this page
See what’s possible with Telmai
Request a demo to see the full power of Telmai’s data observability tool for yourself.
Articles
See all articles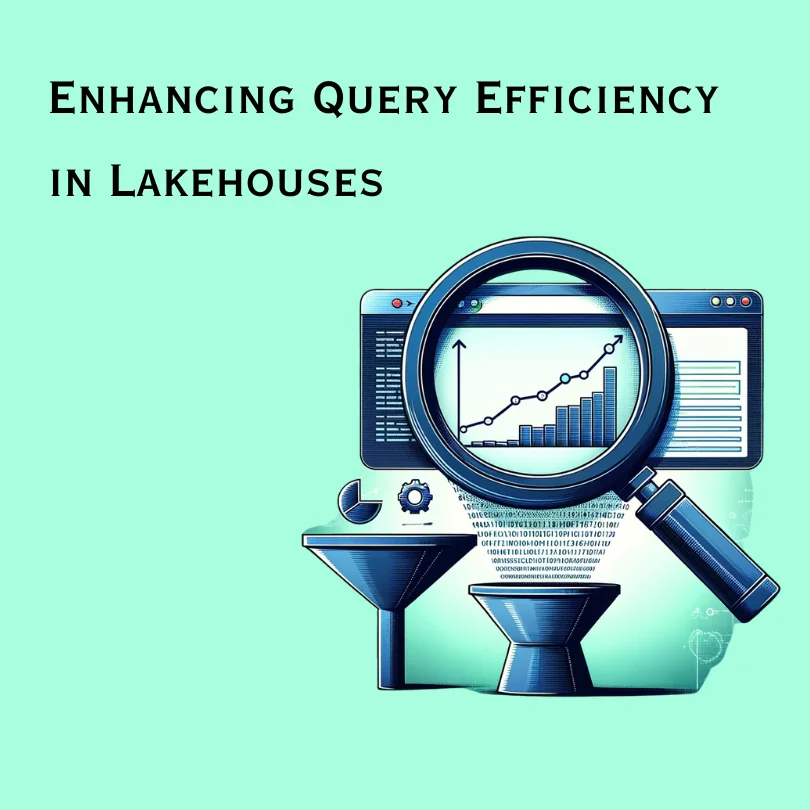