Telmai’s Open Architecture and AI-driven Platform Strengthens Faster Enterprise Adoption
In recent months, we have developed numerous enterprise features that assist data teams in constructing intricate data contracts, decreasing their detection and resolution times for discrepancies in data pipelines, and implementing data observability within their private cloud.
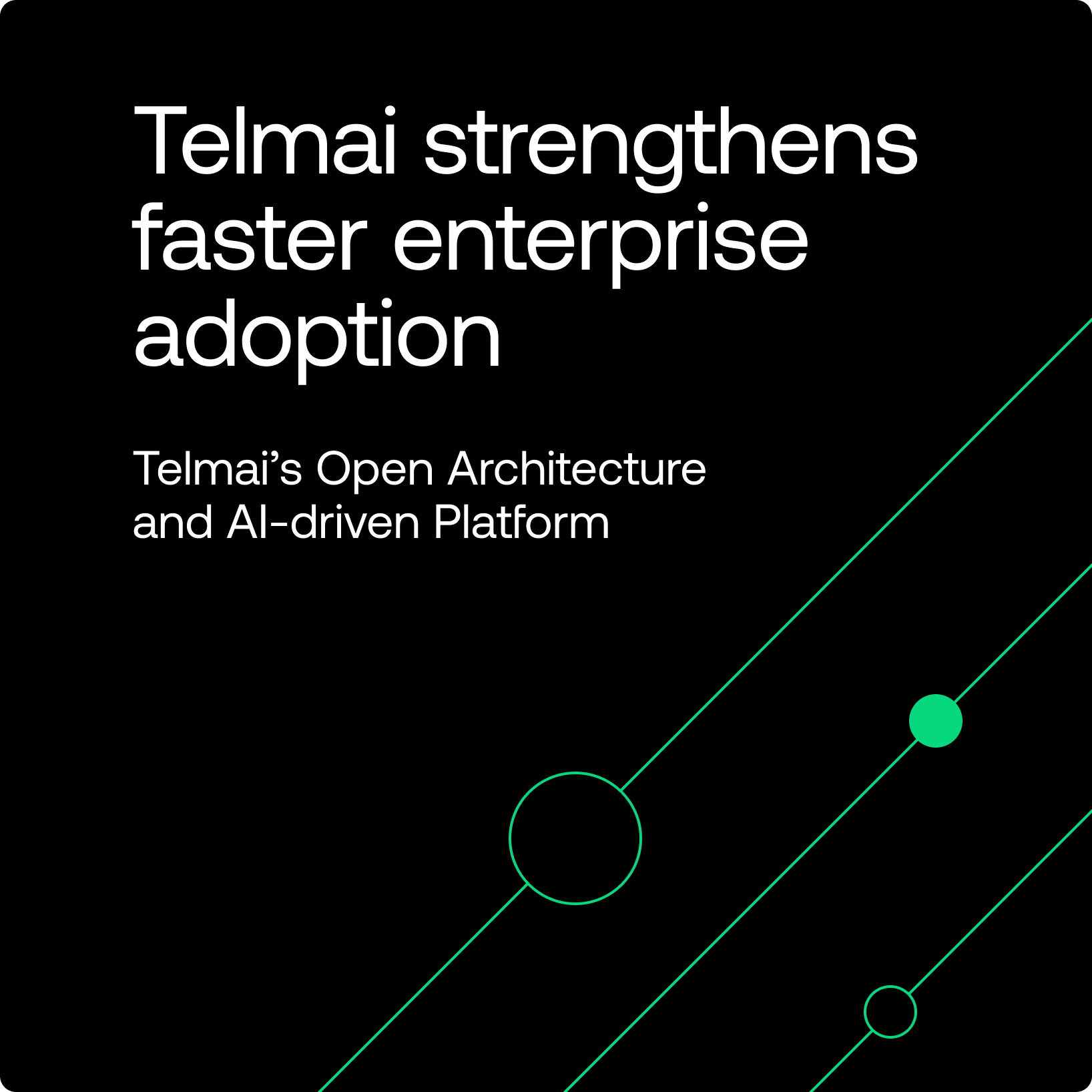
Over the past few months we have created several enterprise capabilities to help data teams build complex data contracts, reduce their time to detect and time to resolve inconsistencies across data pipelines, and deploy data observability in their own private cloud.
These highlights include:
- Multi-Attribute Expectations – Telmai allows defining expressions on groups of attributes and setting expectations on the results. For example, you can track expressions like date_diff that define date_diff (TransactionDate, InvoiceDate) < 7d. These multi-attribute expectations can be defined using familiar SQL-syntax with very efficient calculations to allow for processing of hundreds of expressions over billions of records with minimal performance or cost impact.
- Data Binning – To prevent bad data getting into the downstream systems, Telmai integrates into your data pipeline and splits valid data from invalid based on defined data quality rules. With this feature, you can continue processing good records, while flagging bad records for labeling or cleaning.
- Data Lineage – Telmai captures dependencies between monitored sources and visualizes it in a lineage graph for better root cause analysis of data failures. In addition to the lineage graph, Telmai measures key correlations between dependent sources, detecting when part of the data was lost at a particular step. These consistency checks include volume, uniqueness, and completeness from system to system.
- Metadata Metric Monitoring (beta) – To provide health metrics for tables where deep analysis is not needed, Telmai now offers lightweight analysis of metadata metrics such as record count, table-level freshness, schema changes, and record uniqueness. This helps customers get a set of overall health metrics for any given database, while using Telmai’s deep analysis and data quality investigations for a subset of tables that are more critical for decision making or model accuracy.
- Time Travel Analysis – This feature allows analysis of historical data retrospectively in real time. With this feature, Telmai discovers data anomalies from past weeks, enabling its ML to train on the go, not requiring the system to observe data for some time to come up with predictive outcomes This feature can also accelerate your data quality discovery, policy creation, and testing. By analyzing historical data against new data quality rules, you can in essence “time travel” and see how your data would have performed under policies and business rules you are developing now, helping you gain trust in your data quality formations faster.
- BYOC (Bring Your Own Cloud) for AWS and GCP – Customers can now deploy Telmai in their private cloud to meet data privacy and data residency requirements and manage all cloud costs in one location.
- Google Marketplace Launch – Now customers can leverage their GCP billing including credits to purchase Telmai. This will provide our customers consolidated spending, greater control over costs, faster procurement, and quicker deployments.”
As we continue to add new capabilities and update our platform with your requests, please let us know what is top of mind for you.
To learn more about Telmai, contact me or request a demo.
- On this page
See what’s possible with Telmai
Request a demo to see the full power of Telmai’s data observability tool for yourself.