How Top Data Engineers in Finance Tackle Data Quality Issues
Working in data engineering at a financial company is like walking a tightrope over a pit of regulatory spikes—with the regulators watching through binoculars, munching popcorn, and occasionally shouting “Gotcha!” when someone slips.
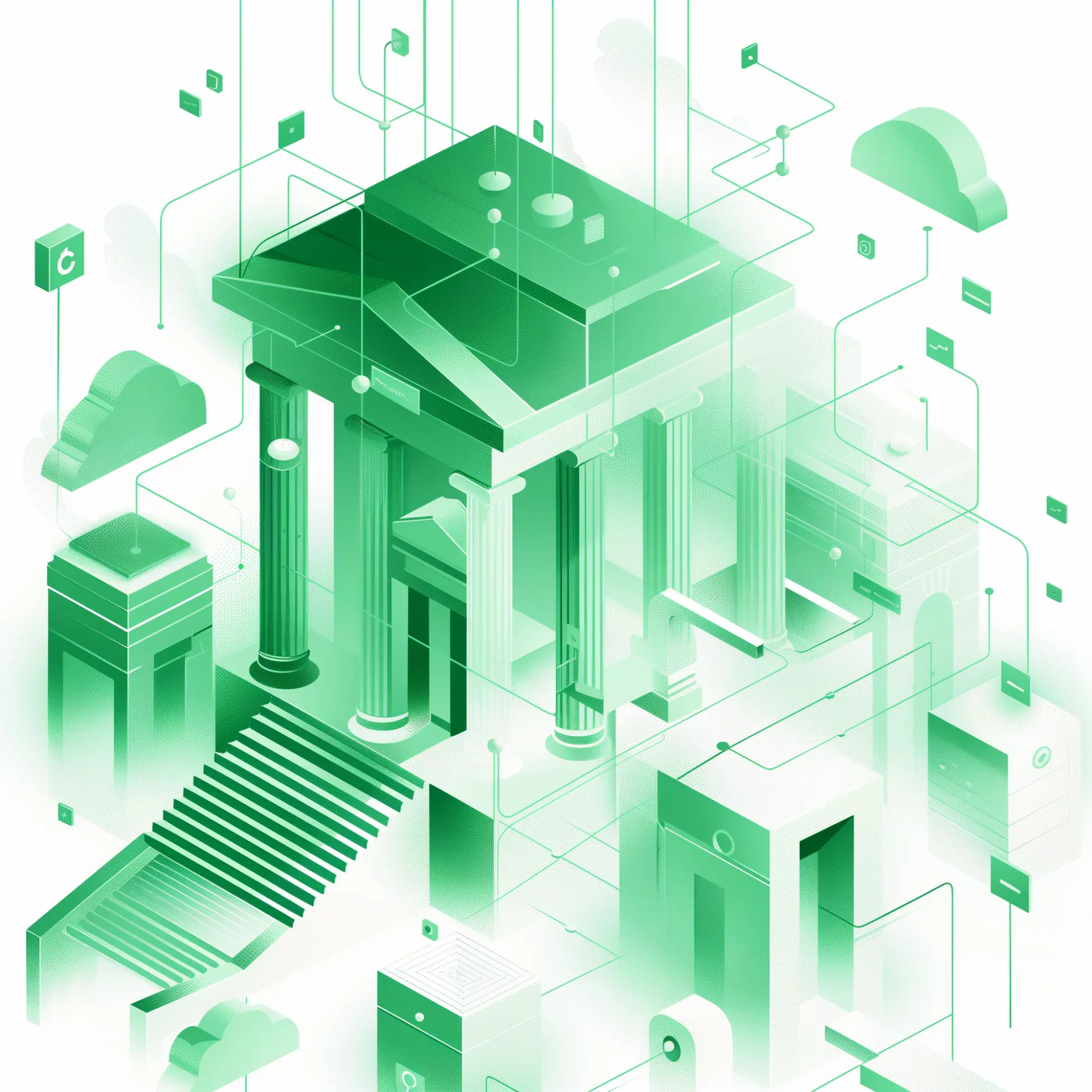
Misplace a digit, mislabel a counterparty identifier, or lazily copy-paste an outdated CCP code, and you’re not just looking at an angry email from compliance, but potentially a multi-million dollar oopsie that makes the front page.
Plus, as we move towards a future filled with more algorithms and AI, the quality of data isn’t just foundational; it’s the very soil from which the next generation of financial technology will grow.
Let’s look at some of the most common data quality challenges financial institutions face and how the best data engineers are navigating them successfully with data observability tool Telmai.
Regulatory Compliance
Accurate reporting to regulatory bodies is the linchpin of adherence to regulations like GDPR, CCAR, and other critical standards such as Basel III, FATCA, and EMIR.
Misreporting can lead to hefty fines and erosion of trust, yet the immense volume of data in the financial services industry renders manual validation and traditional methods impractical and error-prone. Thus, the implementation of robust data quality tools becomes an essential shield against the risks of non-compliance and regulatory scrutiny.
That’s where Telmai comes in. Telmai’s anomaly detection and continuous monitoring of data quality ensure that data inconsistencies are quickly identified and addressed, which is essential for adhering to compliance standards.
Reporting, Analysis, and Forecasting
Data quality serves as the bedrock for reporting activities from routine analysis to intricate risk assessments and forecasting. Inaccuracies here, especially when dealing with vast volumes of real-time data, can lead not only to flawed risk calculations but can also skew the strategic forecasts that guide future investments and policy decisions.
Telmai provides complete pipeline visibility and cross-system consistency – regardless of the type, form, shape, frequency, and volume of data in the pipeline – to support more precise forecasting and more informed decision-making. Better still, Telmai does all this on a dedicated compute layer separate from your data warehouse or analytical databases to prevent the overload of your systems.
Obtaining High Quality Data for Training AI models
The quality of data used to train AI models determines the quality of insights that financial models can offer. Poor data quality is akin to feeding incorrect coordinates into a navigation system—the results are misleading directions that could potentially lead off a cliff.
Telmai helps to reduce the cost and timeframe of bad quality data impacting AI training. See our blog post Demystifying Data Quality’s Impact on Large Language Models where we deliberately introduce errors into the data used to fine-tune a LLM and discuss strategies and practices for ensuring high-quality data.
Data Governance
Without data governance, financial institutions risk facing unchecked data proliferation, compromised data quality, more security vulnerabilities, and potential non-compliance with regulatory standards, ultimately leading to poor decision-making and reduced efficiency.
Data governance policies help solve these challenges by defining roles and responsibilities for data management. For example, data ownership, data access, and data security policies.
With over 250+ integrations, Telmai ensures that data governance policies can be uniformly applied across various data sources and systems, while providing data lineage tools to track the changes and transformations.
Cloud Migrations
Imagine a financial institution that has grown over the years through mergers and acquisitions. They’re now consolidating customer data from multiple legacy systems (each with their own data format, quality standards, and levels of completeness) into a new, unified system. They ensure data integrity during these migrations by double-checking the source and target data to make sure nothing is lost or altered in the journey.
Telmai simplifies migrations and reduces risks by automating data profiling. Data profiling speeds up the design and development of the migration by identifying all of the transformations and data cleansing activities required to transition the data safely.
Maintain data quality excellence with Telmai
The complexity and volume of data in finance today demand not just vigilance but automation. Employing automated data quality solutions not only streamlines the maintenance of data quality but elevates it, allowing banks and financial institutions to not just react to data issues but anticipate and prevent them. This technological leap forward is where solutions like Telmai come into play.
Telmai’s edge lies in its user-friendly interface and the deployment of AI-driven analytics, utilizing advanced machine learning algorithms to foresee and proactively address potential data quality issues. With its intuitive dashboard, users can effortlessly visualize data health, trace lineage, and understand quality metrics, empowering financial institutions to operate with the highest data integrity and analytical insight.
Take a proactive approach to mitigate risks and ensure accuracy in financial operations. Request a demo of Telmai today.
- On this page
See what’s possible with Telmai
Request a demo to see the full power of Telmai’s data observability tool for yourself.