How to maintain data quality
Select the right data quality indicators, set monitoring thresholds, and address any data deviations. It’s easier said than done, but with the right processes and data quality tools it’s possible to transform data quality management from a reactive to a proactive stance.
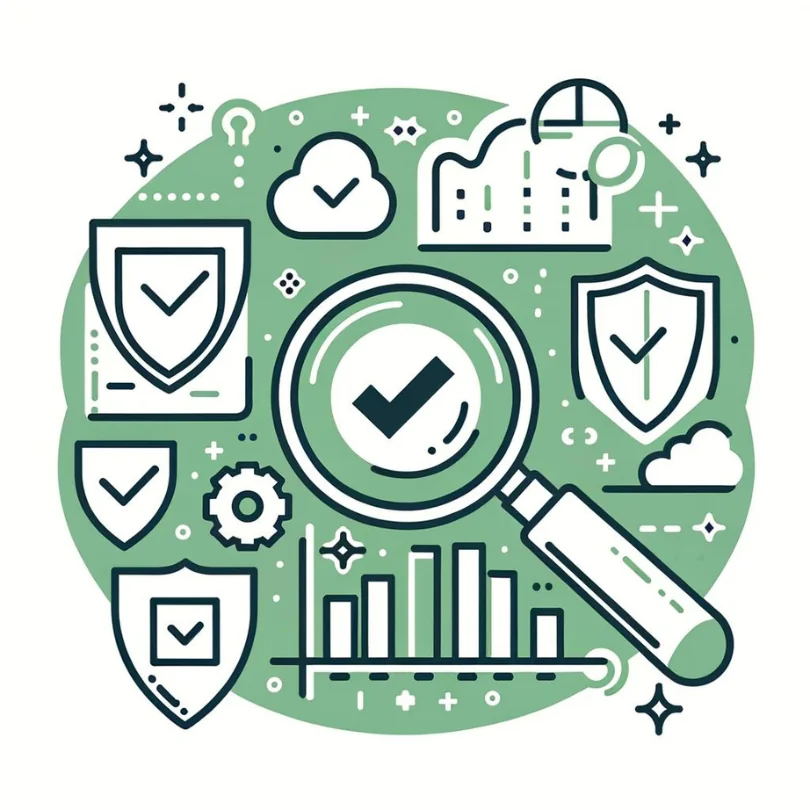
Our 2024 State of Data Quality survey revealed that the most common way to maintain data quality, as reported by 27% of respondents, was with a homegrown solution. This was followed by 20% who rely on traditional data quality tools like Informatica and Collibra and 16% who indicated that they do not have a specific solution in place at all.
None of these ways is the best way to maintain data quality.
Instead, follow the steps below and learn how organizations that use a modern data stack are using modern data quality tools to save time and improve data quality.
1. Establish data quality indicators
Maintaining data quality starts with selecting the right data quality indicators. Your indicators should align with your business goals.
2. Implement data validation rules
The right data validation rules ensure that only valid data is entered into your system. For example, use drop-down lists, checkboxes, and other data entry controls to prevent data entry errors.
To detect anomalies or outliers that do not violate any explicit rules, use a machine learning-based data observability tool like Telmai, which learns the normal behavior of your data and detects anomalies and outliers that might indicate unknown or unexpected data quality issues.
3. Profile and track data as it moves
Profile your data and identify any inconsistencies, duplicates, or missing values. These are areas where you need to improve your data quality.
Tools like Telmai can profile and inspect your data at scale, with interactive visualizations and comparison with historical insights.
4. Implement data cleansing processes
Implement data cleansing processes to remove duplicates, correct errors, and standardize data formats. This includes using data matching algorithms, data normalization, and data standardization techniques.
5. Monitor data quality
Continuously monitor your data quality by tracking your data quality indicators over time. Use data quality dashboards to visualize your indicators and identify areas for improvement.
Telmai’s investigator provides an easy-to-use, no-code interface, and integrations with ticketing solutions like Jira allow your team to take action faster.
6. Train your staff
Train your staff on the importance of data quality and how to maintain it. This includes providing training on data entry best practices, data validation rules, and data quality standards.
7. Establish data governance policies
Establish data governance policies that define roles and responsibilities for data management. For example, data ownership, data access, and data security policies.
8. Use a modern data quality tool like Telmai
If your organization uses a modern data stack, you should use a modern data quality tool like Telmai.
Telmai stands out for its rapid deployment, ease of maintenance, and intelligent, always-on monitoring that delivers insights swiftly. As an AI-based data observability platform designed for open architecture, Telmai excels in real-time monitoring across the data pipeline, from data warehouses to cloud storage systems. It proactively detects anomalies in both structured and semi-structured data sources, ensuring decisions are based on reliable data and preventing bad data from impacting critical decisions.
Discover how Telmai can revolutionize your data ecosystem. Request a demo today.
Passionate about data quality? Get expert insights and guides delivered straight to your inbox – click here to subscribe to our newsletter now.
- On this page
See what’s possible with Telmai
Request a demo to see the full power of Telmai’s data observability tool for yourself.