9 Steps to Ensure Data Quality at Scale
Maintaining the quality of data is a meticulous journey that requires careful navigation. Overlooking steps can jeopardize your insights, while overdoing it can hinder swift decision-making.
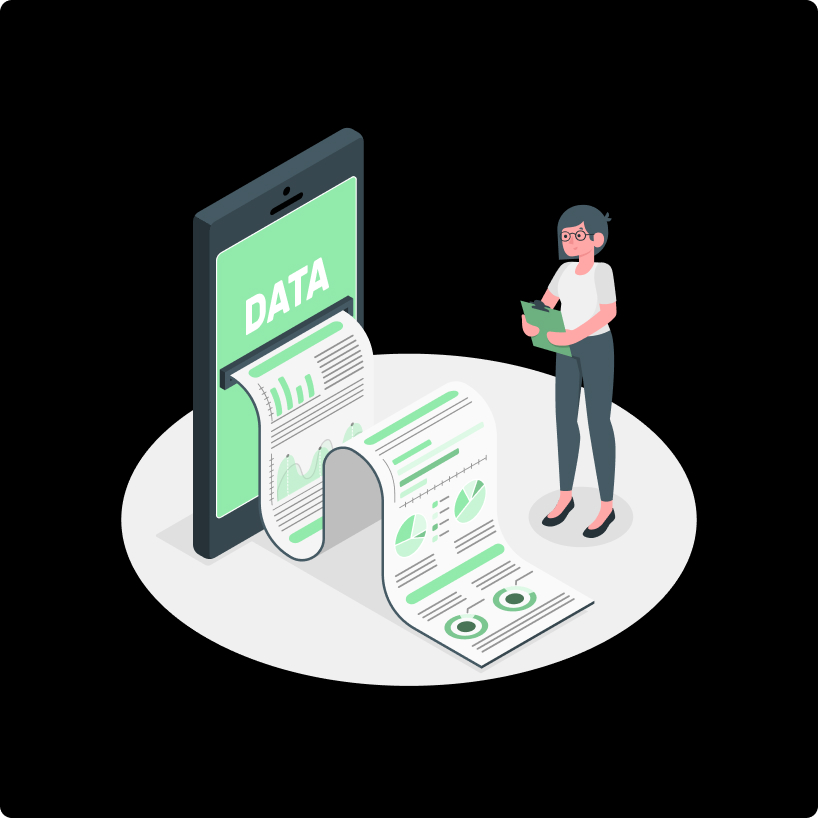
Ensuring data quality is a systematic process that involves multiple steps. Skip too many and the integrity of your insights becomes compromised, yet overcomplicate measures and you delay decision-making.
To strike a balance that achieves trust in data without reducing the agility of business operations, you can’t go wrong with the process below.
Why ensure data quality?
Data quality refers to the accuracy, reliability, completeness, and consistency of data. It is the process of optimizing data to align with the desired state and meet the expectations defined in the business metadata.
In essence, data quality answers the fundamental question, “What do you get compared with what you expect?”
Ensuring data quality is of paramount importance because it forms the foundation for informed decision-making, accurate analysis, and effective business strategies. High-quality data instills confidence in the insights derived from data-driven processes, leading to better outcomes and a competitive edge in the market.
1. Define clear data quality standards
Standards serve as the benchmark against which data quality is measured, and help in identifying areas of improvement. Here’s how to go about it:
- Identify Critical Data Elements (CDEs) crucial for your business operations and decision-making.
- Set permissible levels for data quality indicators like accuracy, completeness, and consistency for each CDE.
- Develop metrics to gauge the quality of data against the defined standards, such as error rates and duplicate data.
- Involve stakeholders in defining these standards to ensure a holistic approach and gain valuable operational insights.
- Document the standards to maintain a consistent understanding and execution across the organization.
By establishing clear standards, you create a shared understanding of what constitutes “good” data within your organization, setting the stage for all subsequent data quality management activities.
2. Establish a data governance framework
Now that you have defined your standards, establishing a data governance framework is the next step. A framework ensures that data throughout your organization is handled consistently, roles and responsibilities are clearly defined, and data-related issues are addressed promptly and effectively.
- Allocate roles and responsibilities for data governance to ensure accountability.
- Formulate clear policies and procedures for data handling, usage, and quality assurance.
- Designate data stewards to oversee data quality and compliance within different business units.
- Establish a council with representatives from key business areas to guide data governance strategy and resolve issues.
- Employ data governance tools to automate processes, monitor data quality, and ensure compliance. The data observability platform Telmai can do this and more.
- Foster a culture of data quality through training and awareness programs.
- Establish metrics to measure governance effectiveness and ensure continuous improvement. For example, policy compliance rate, issue resolution time, and stakeholder satisfaction levels.
By putting a robust data governance framework in place, you lay a solid foundation for maintaining data quality, ensuring compliance, and fostering a culture of data excellence.
3. Profile existing data
Now that a structured governance framework is in place, profiling your data is crucial to understand its current state and quality levels. Follow these steps:
- Understand the structure of your data including the data types, relationships, and patterns within it.
- Assess the quality of your data by evaluating key indicators such as inconsistencies, missing values, and deviation from established benchmarks.
- Document your findings to provide a baseline for improvement efforts, capturing issues that may affect data quality.
- Leverage data profiling tools to automate the analysis and gain deeper insights into your data quality. Again, Telmai can help here.
By profiling your existing data, you gain a clear understanding of its strengths and weaknesses, which is instrumental in planning and prioritizing data quality improvement initiatives.
4. Validate incoming data
With a solid understanding of your existing data, the next step is to ensure that all new, incoming data aligns with your data quality standards. Take this approach:
- Establish rules based on your data quality standards to validate the accuracy, consistency, and completeness of incoming data.
- Utilize the data observability tool Telmai to automate the checking of incoming data against your established rules.
- Identify and log errors for further analysis and remediation.
- Create a feedback loop with data providers to communicate errors and ensure continuous improvement in data quality.
- Continuously monitor the validation process to identify trends, improve validation rules, and ensure data quality is maintained over time.
By validating incoming data, you ensure that it meets the predefined standards before entering your systems, thereby maintaining a high level of data quality and minimizing the risk of errors.
5. Cleanse data regularly
No validation process is foolproof. There could be errors that slip through the cracks and are only discovered later, business requirements or data standards may change, inconsistencies may arise when integrating new data sources or merging databases, or data can simply become outdated or irrelevant over time, a phenomenon known as data decay.
Regular data cleansing is essential to maintain high-quality data. Employ automated cleansing processes using tools like Telmai to correct or remove erroneous data. With Telmai you can monitor data continuously to identify issues or anomalies in real-time and receive an alert or run remediation actions automatically.
6. Conduct regular audits
Traditionally, regular audits have been a cornerstone in maintaining data quality. Audits entail a systematic examination of how data is collected, stored, managed, and used to ensure adherence to established data quality standards. They help in identifying inconsistencies, errors, and deviations that could adversely impact decision-making and compliance.
However, with the advent of data observability tools, the paradigm is shifting towards a more proactive and continuous approach to ensuring data quality.
The automation and real-time nature of data observability tools like Telmai make them more efficient compared to the scheduled and manual process of traditional audits.
Learn how Telmai automates the monitoring processes, reducing the manual effort required in traditional audits, and minimizing the risk of human error.
7. Train staff
Promoting a culture of continuous learning is crucial for staying updated with evolving data quality standards and technologies. Provide access to ongoing training resources, workshops, and seminars to encourage continuous learning. Regularly assess the skills of your staff regarding data management and data governance to identify areas where training is needed.
8. Document processes
You’ve got a bunch of processes – collecting data, validating it, integrating it, monitoring it, and managing it. Now, what? Document them. Lay it all out—step by step, tool by tool, who does what, and when. Make it clear, make it accessible. Use diagrams if you have to—whatever it takes to make complex processes digestible. And while you’re at it, keep it consistent; a standard format across all documentation is your friend here.
Now keep it updated. Processes evolve, tools get upgraded, and standards shift. Your documentation should keep up with the times. Get the folks who own the processes involved; they know the ins and outs.
Good documentation holds everything else together, supporting data quality management, compliance, and a culture of continuous improvement.
9. Refine and improve processes
So, you’ve got your processes documented, everyone’s trained up, and things are ticking along. Now what? Sit back and relax? Not quite. Welcome to the never-ending saga of refining and improving processes. It’s like tuning a vintage car; there’s always something to tweak to get that extra purr. Your data quality processes are no different.
Dive into the metrics, analyze the outcomes, and compare them to the goals. Are there gaps? Great, you’ve got work to do. No gaps? Look harder. There’s always room for improvement.
Adapt processes based on the feedback and the metrics, test the changes, and measure the impact. Rinse and repeat. It’s a cycle of continuous improvement—measure, refine, implement, measure again. And while you’re at it, loop back to documenting these refinements; keep that organizational memory fresh and updated.
Ready to take the next step in your data quality journey? Try Telmai
Ensuring data quality is not just a one-time task; it’s an ongoing process that requires continuous monitoring and control.
Imagine a world where data discrepancies wave a red flag before they morph into monstrous problems. That’s Telmai for you.
With Telmai, you’re not just reacting to data issues; you’re staying ahead of them. Request a demo, explore its features, and see how it elevates your data quality game.
- On this page
See what’s possible with Telmai
Request a demo to see the full power of Telmai’s data observability tool for yourself.