Data Profiling for your Data Warehouses and Data Lakes
Cloud migration and integration projects hinge on high-quality data, yet traditional technologies falter in managing the volume and complexity of modern cloud computing. This article delves into a case study where an organization incorporated next-gen cloud data quality technology into its delivery approach, resulting in superior projects in less time and paving the way for future project innovation.
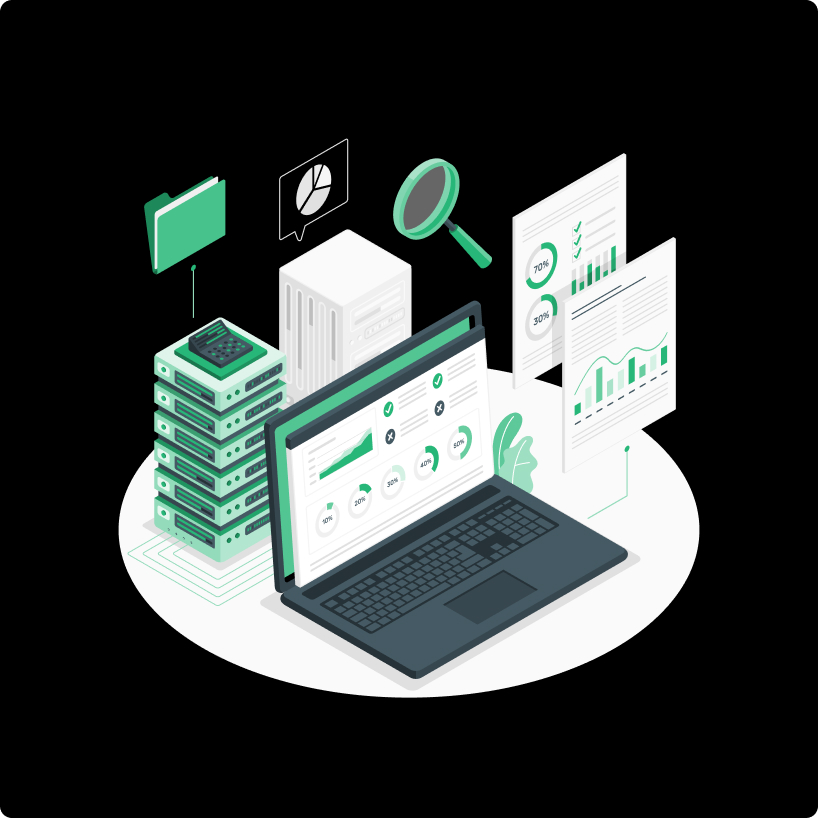
Cloud migration and integration projects rely on good quality data to meet their objectives. However, traditional technologies have struggled to manage the volume and complexity of modern cloud computing and storage.
This article explores a use case from one organisation that embraced the next era of cloud data quality technology by incorporating it directly into its delivery approach.
The outcome has delivered better quality projects at a fraction of the time than conventional approaches whilst opening up exciting avenues for future project innovation.
The demands of cloud-based data profiling
Myers-Holum, Inc. (MHI) operate at the cutting-edge of data engineering and data integration, having helped over 1000 enterprises to streamline their operations, financials and business processes.
Driven by a desire to reduce maintenance costs, increase computing power, and exploit the massive rise in data volumes, clients reach out to MHI for help in either transitioning legacy analytical systems into Google Cloud or building out entirely new Google Cloud analytical solutions.
MHI, therefore, has a compelling need to understand the data structures, content, and relationships within client system data to prevent data anomalies or defects from going undetected when migrating to Google Cloud as well as shaping the design of the target platform.
This discovery exercise is what is commonly referred to as ‘data profiling’.
Data profiling forms a core component of data management and is crucial to the many complex data engineering initiatives that specialist data firms like MHI undertake.
The typical client of MHI is looking to analyse billions of data elements from sources such as:
- Google, Facebook ad networks
- In-app event streams
- Finance market event data (such as Bloomberg)
- Corporate sales and finance data
Data profiling speeds up the design and development of the analytical cloud platform solutions that will leverage this source data whilst identifying all of the transformations and data cleansing activities required to transition the data safely.
But despite a busy data profiling vendor marketplace, MHI had struggled to find a traditional profiling solution that could cope with the evolution of data volumes and processing performance typified by cloud computing (as well as work well with the new cloud technology stack).
The tools they initially tested originated from the previous ‘on-prem’ data management and engineering era, where volumes and compute speed were far lower. These legacy tools left MHI with a gap when attempting to scale data profiling economically and reliably for a cloud-based technical stack.
Darius Kemeklis, EVP of the MHI Google Cloud Practice, explains:
“We had grappled with the existing data profiling technologies for some time. Many of the tools were limited in their outputs and analytics, making it impossible to share insights with clients.
But the biggest challenge was scale.
Either the legacy profiling architectures meant we were forced to rely on sampling (which didn’t address our needs), or they were too cumbersome to support a consulting workflow that requires the analysis of thousands of attributes and billions of data points”.
Given these limitations, Telmai began working with Darius’ team at MHI to leverage the Telmai cloud-based data profiling and quality solution to improve their cloud migration workflow and reduce the cost/timescales of hand-cranked data profiling activities.
Incorporating data profiling into the data migration consulting workflow
Companies such as MHI and others realise that when building integration and migration processes, you can’t move to the next phase of designing and engineering data pipelines until the data profiling and quality assessment work has been completed.
For example, you can’t build mapping and transformation rules between source and target data stores without understanding:
- Detailed structural schema analysis
- Data quality analysis to identify data content problem and risk areas
- Distribution of data values and patterns to identify the different standards and rules inherent to the data
- Redundant attributes that are either empty/incomplete, or have not been maintained
Skipping these phases dramatically increases the likelihood of failure during a data migration and integration project.
During a recent Telmai interview with Dylan Jones (editor of Data Quality Pro and Data Migration Pro), Dylan explained the research findings that linked data profiling to successful data migration outcomes:
Telmai: How has data profiling improved data migration outcomes?
Dylan Jones: It’s hard to understate how big a shift we’ve seen due to improved technologies and practices in data profiling / data quality management.
Historically, data migration projects were high-risk ventures.
Back in 2007, only 16% of data migration projects came in on time and under budget. Given the high failure rate, data migration was thought of as a ‘poisoned chalice’ if you were in charge of delivery.
But when we researched the industry in 2017, we found that 60% of projects were considered successful.
The adoption of data profiling heavily influenced that success.
For example, the 2007 research showed that only 10% of projects used data profiling tools. But by 2017, the adoption of data profiling tools had risen to 70% (in the US) and is even higher today. There’s a clear link between data profiling, data quality and project outcomes.”
The link between data profiling and accurate project forecasting
One of the biggest challenges that data profiling addresses is helping to scope and assess the risks associated with a data migration or integration project.
It’s impossible to determine how complex or costly the project will be for integration partners and customers without accurately assessing the legacy data sources and the migration path they need to take before reaching the target system.
During the same interview, Dylan Jones expanded on this scoping challenge and its dependency on data profiling:
Telmai: How can data profiling influence the project scoping and forecasting analysis of a complex migration?
Dylan Jones: “One of the reasons many projects still come in over budget or blow their delivery timescales is they lack an effective forecasting strategy that is driven by reality as opposed to guesswork and misplaced assumptions.
For example, our research observed that 50% of projects lacked an effective forecasting and scoping strategy.
Today, the challenge is compounded because so many migration projects are cloud-related, which means the volumes and complexity of data sources is significantly higher than ever before – greatly increasing the risk of budget and timescale increases if the forecasting is flawed.
The key is to undertake a Pre-Migration Impact Assessment, which is a fancy way of saying – profile your data extensively!
You must understand the data structure, content, and quality before committing investment and planning for your migration.
By profiling your data in advance, you’ll have a clearer understanding of the pitfalls that await you, the skills and resources you’re going to need and the likely duration and complexity of the project.”
How does data profiling feature throughout the rest of the data migration?
We’ve highlighted the importance of data profiling at the outset of an integration/migration project – but how can it be applied after this?
Drawing again on the MHI use case, we can see how data profiling can support the design of the target solution by sanity checking the quality and content of event data.
Darius Kemeklis explains:
“For many of our clients, we need to process and analyse large amounts of event data. An example could be a client with money transfer systems that record vast quantities of interaction data with its customers.
By understanding this data, we can model the totality of user engagement. This knowledge helps us build the right data analytics and warehousing solution to optimise each client’s marketing campaign and transaction performance.
Data profiling plays a critical role because it helps us quickly cut through the noise to identify the most vital information and assess its fitness against the intended purpose of the target system.”
So, this kind of use case demonstrates how cloud-based data profiling is accelerating and shaping target system design due to its ability to assess and report on event data volumes that would have been inconceivable with legacy profiling technologies.
Beyond the data migration – how can cloud-based data profiling play a role after go-live?
At Telmai, we’re excited about the implications of cloud computing on the type of data services that solution providers and their clients can support after the migration.
When the migration or integration is finished and the target system goes live, the data quality assurance work is not complete – in fact, it’s just getting started.
Systems and applications constantly adapt to the subtle shifts in business models, consumer needs, and competitive pressures shaping application and data design.
If unchecked, data quality defects will continue to occur, both as a result of system and user behaviour changes, as well as the inherent failure rate that massive volumes of data and user interaction inevitably creates.
The ability to measure and monitor data quality remotely was not lost on MHI following their recent shift to cloud-based data profiling with Telmai.
As Darius Kemeklis explains:
“Historically, we would deliver the final migration and hand everything over to the customer. But the challenge is what happens if something like an industry coding standard changes or a particular user starts entering information in a different format?
By applying these new approaches to cloud-based data profiling and data quality monitoring, we’ll be able to build alerts that instantly target and report defective data before it leads to problems.
This allows our customers to proactively monitor data quality instead of relying on the business users to notify the technology teams when the data goes bad.”
There are many ways that management reports, analytics and operational processes can become defective after the migration if the underlying data quality isn’t continuously assessed and monitored.
By building up an earlier profile of the target data that you know to be correct, you can build a 24/7 operational data quality reporting platform by leveraging the data profiling and data quality rules, technologies, and processes delivered during the migration.
With the flexible and remote capabilities offered by cloud computing, this means it’s easier than ever to deliver these types of value-added data quality services.
Summary and next steps
Cloud migration and integration projects benefit significantly from data profiling and data quality interventions, but for many years the sheer scale and volume of cloud computing created a barrier to traditional data profiling technologies.
The case story we present in this article introduces the next generation of cloud-based profiling solutions and their potential, now and in the future.
If you would like to personally experience a demonstration of how Telmai can help improve the quality and outcome of your cloud migration or integration project, then reserve your demonstration below:
Book a demo now and see for yourself.
- On this page
See what’s possible with Telmai
Request a demo to see the full power of Telmai’s data observability tool for yourself.