Unlocking Data Potential: The Power of Merging Data Catalogs and Observability
In the rapidly evolving digital world, data professionals navigate complex challenges posed by advancements in Artificial Intelligence (AI) and Machine Learning (ML). The advent of Data Catalog and Observability tools marks a transformative phase in data management, offering structured visibility, real-time monitoring, and actionable intelligence to address the fundamental challenges faced by data engineers, analysts, and scientists.
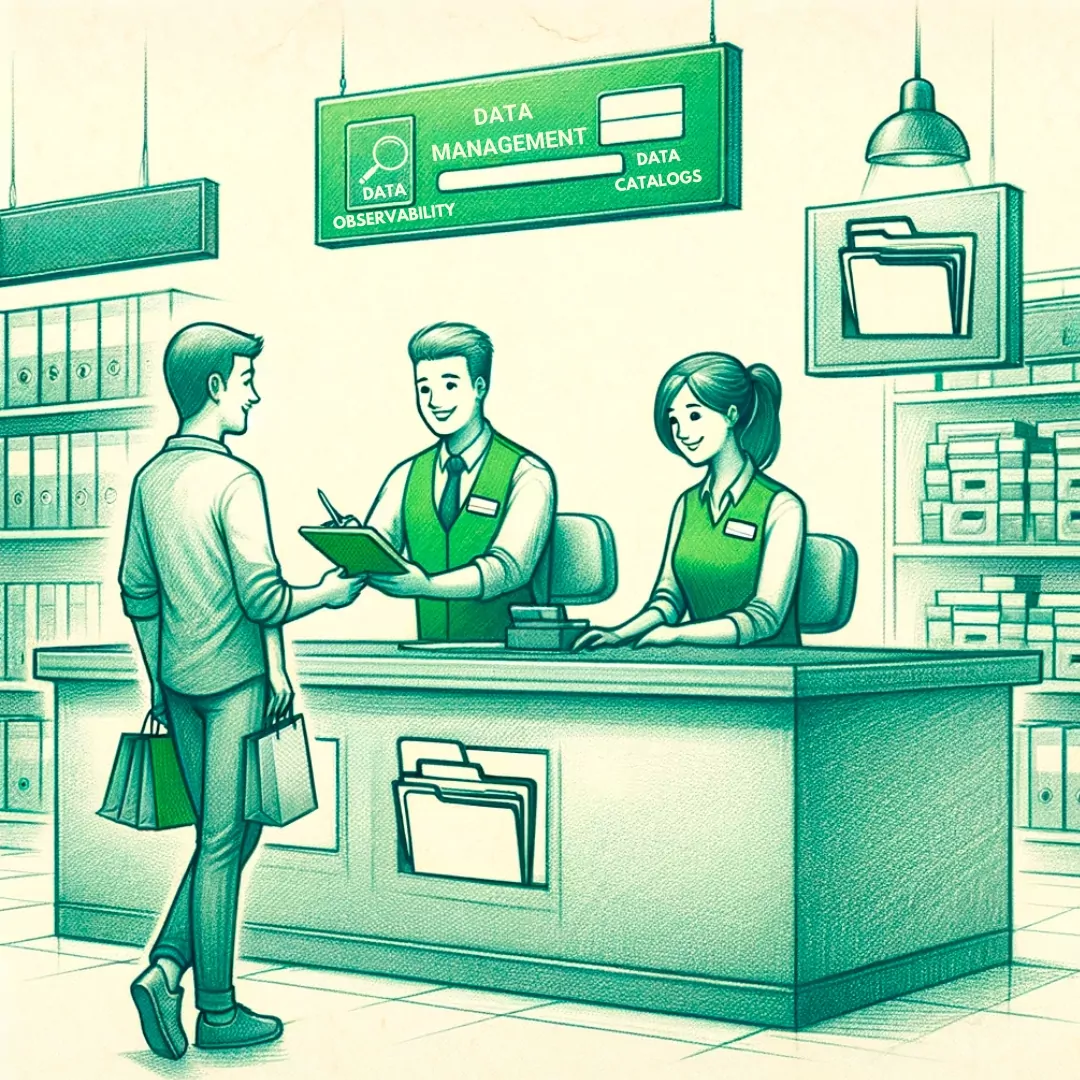
In today’s fast-paced digital world, which is constantly reshaped by the advancements in Artificial Intelligence (AI) and Machine Learning (ML), picture the life of a data professional who navigates through these complex and ever-evolving challenges.
Data engineers frequently encounter challenges in managing data flow and processing, primarily due to a lack of clear information about the data’s origin, structure, and lineage. This ambiguity hampers their ability to construct and maintain data pipelines effectively. On the other hand, data analysts often grapple with ensuring data accuracy and consistency. Their challenges also include comprehending the context of data sets and verifying the currency and relevance of the data they utilize for analysis. Data scientists, meanwhile, face obstacles in identifying meaningful patterns and trends. Irregularities or gaps in historical data often complicate their work, and they may struggle to replicate experiments or comprehend the impact of changes in data sources on their models.
Data Catalog and Observability tools mark a transformative phase in data management. These tools bring structured visibility and real-time monitoring to the entire data lifecycle. They offer clear mapping, lineage tracking, and quality insights, directly addressing the fundamental challenges faced by data engineers, analysts, scientists, and other data professionals. This shift ushers in an era of clarity and actionable intelligence, replacing potential disarray with systematic and informed understanding.
What is a Data Catalog?
Imagine a supermarket with rows of produce, each item offering different flavors and nutrients. Finding the specific fruit or vegetable you need can be challenging, especially if you’re unfamiliar with its appearance or name. This is where labels and signs in the supermarket come in handy, guiding you to the right section and providing information about each item.
A data catalog serves a similar purpose for organizations with vast data. Just as labels in a supermarket help you navigate through different aisles and identify various products, a data catalog helps navigate large volumes of data. It is a centralized metadata repository providing detailed information about the organization’s data assets. Think of it as the labeling system that organizes and categorizes your data, making it easily searchable and accessible.
A data catalog goes beyond simply listing data sources. It enriches the data with descriptive details, such as its origin, format, lineage, quality metrics, and usage patterns. This wealth of information makes it easier for data professionals to discover, understand, and trust the data they need. This way, everyone can be more productive and make better decisions because they have the correct information.
What is Data Observability?
Now, think about our supermarket again. You wouldn’t pick a can of milk that is expired? Data observability is like a vigilant store assistant who continuously inspects the produce. They ensure that each item is fresh (taking note of expiry dates) and correctly sourced, highlighting its origin, whether locally grown or genetically modified.
For businesses, data observability is about fully understanding the state of data in the system. It’s like a health check, detecting and diagnosing issues with data quality, pipeline failures, schema changes, and other anomalies in real-time. It keeps track of data health throughout its lifecycle, ensuring accuracy, consistency, and reliability.
Convergence of Data Catalogs & Data Observability
Returning to the analogy, when you combine the informative labels and signs (data catalog) with the attentive store assistant (data observability), you achieve an exemplary supermarket experience. You can swiftly locate the correct item, fully informed of its source, whether it’s local or genetically modified, and its freshness. This ensures that your selection meets your expectations – fresh, ripe, and accurately represented, with no surprises in quality or origin.
Data observability often has limited visibility in the business context of data assets, while data catalogs may not have real-time insights into data quality and performance issues. Moreover, data producers and consumers may need to switch between multiple tools to get a holistic view of their data, which can be time-consuming and inefficient.
Combining these two makes sure that businesses can find their information quickly and trust that the data is good. That way, when they have to do important stuff, like figuring out what new products to make or what customers to target, they make those choices based on the best and most accurate info they have. It’s like having a super-smart supermarket that’s automatically organized and reliable!
Data catalogs and observability serve complementary functions—one is about organizing and understanding data assets, and the other is about monitoring and ensuring data quality and pipeline health. There’s a natural synergy between them, as a data catalog enriched with observability insights provides a more comprehensive understanding of an organization’s data assets and reliability.
Who Benefits From This Union?
The convergence of data catalog and observability can lead to the democratization of data across an organization, where each persona benefits from the insights (See Table 1). Here are some of the benefits of a unified approach:
- Enhanced Data Governance and Remediation: For roles like Data Engineers and Data Stewards, the convergence of Data Catalog and Observability tools facilitates quicker resolution of data issues and better data governance by linking real-time monitoring with comprehensive data knowledge.
- Streamlined DataOps and MLOps: Roles such as Data Analysts, ML Engineers, MLOps Engineers, and Data Scientists benefit from streamlined operations and improved workflow efficiency, as they can quickly identify and resolve data issues, ensuring high performance of models and analysis.
- Integrated Insights for Strategic Planning: For Data Architects, IT Operations Managers, and ML Product Managers, this convergence aligns data strategy with actual data health, enabling more informed decisions and strategies, particularly regarding data infrastructure, compliance, and product development.
# | Persona | Key Tasks | Benefits from Data Catalog | Benefits from Data Observability | Benefits from Convergence |
1 | Data Engineer | 1. Building and managing ETL pipelines.2. Ensuring data quality.3. Data modeling and warehousing. | Uses to document data lineage and metadata, facilitating easier integration and troubleshooting. | Monitors the health of data pipelines, ensures data quality, and identifies issues in real time. | Can trace issues directly from the observability tool to the catalog for quick resolution and better data governance. |
2 | Data Architect | 1. Designing data models and databases.2. Data strategy development.3. Overseeing data management infrastructure. | Relies on catalogs to understand existing data assets and how they can be integrated into new solutions. | Uses observability to assess the performance and scalability of the data infrastructure. | Better alignment between data strategy and actual data health, enabling more informed architectural decisions. |
3 | IT Operations Manager | 1. Managing IT resources and infrastructure.2. Ensuring system reliability.3. Implementing IT policies and security. | Data catalogs help in managing data assets as part of the overall IT asset management. | Data observability is key for ensuring the operational health of data systems and infrastructure. | Improved incident response through integrated data health insights and asset information. |
4 | Data Steward | 1. Data governance and compliance.2. Data quality control.3. Metadata management. | Central to their role in maintaining an organized, searchable, and governed data ecosystem. | Used to monitor compliance and data quality across the system, ensuring standards are met. | A combined view helps maintain higher data quality and compliance with less manual intervention. |
5 | ML Engineer | 1. Designing and developing ML models.2. Deploying models into production.3. Model performance tuning. | Locates and understands datasets for training models, including features and labels. | Monitors model performance and data drift to ensure models remain accurate over time. | Quick identification and resolution of data issues that affect model performance. |
6 | MLOps Engineer | 1. Automating ML pipelines.2. Ensuring ML model scalability.3. Monitoring model deployment. | Utilizes catalog for versioning and tracking dependencies in ML pipelines. | Uses observability to ensure the deployed models are functioning as expected in production. | Streamlined operations with faster issue resolution and model management. |
7 | Application Engineer | 1. Integrating ML models into application platforms.2. Ensuring application scalability and performance.3. Troubleshooting and debugging applications. | Uses the catalog to understand how data flows into and out of ML models for better integration. | Leverages observability to monitor application performance and quickly address any model-related issues. | Faster and more efficient debugging and maintenance of ML-powered applications with combined data insight and tracking. |
8 | Generative AI Developer | 1. Designing and training generative AI models.2. Experimenting with model architectures.3. Adapting models for various applications. | Utilizes the catalog to source and manage datasets for model training. | Observes model behavior in different conditions for performance tuning. | Streamlined model development with combined dataset management and monitoring. |
9 | AI Ethicist | 1. Assessing ethical implications of AI.2. Developing responsible AI guidelines.3. Advising on AI governance. | Uses the catalog to trace data lineage and ensure ethical sourcing. | Monitors data usage patterns for ethical compliance. | Enhanced accountability and ethical oversight with linked data lineage and usage. |
10 | Data Analyst | 1. Analyzing large datasets to extract actionable insights.2. Creating reports and visualizations.3. Collaborating with business teams to inform decision-making. | Utilizes the data catalog to find relevant and reliable data sources. | Employs data observability to ensure the accuracy and timeliness of data. | Enhanced analytical capabilities through integrated access to quality-assured and up-to-date data sources. |
11 | Data Scientist | 1. Analyzing data for insights.2. Developing predictive models.3. Interpreting data to inform business strategies. | Finds and understands datasets to speed up analysis and modeling tasks. | Ensures datasets for model training are accurate and reliable for high model performance. | Streamlined access to reliable data (observability) and metadata (catalog) can greatly enhance workflow efficiency. |
12 | ML Product Manager | 1. Defining product vision and strategy.2. Coordinating cross-functional development.3. Overseeing go-to-market and user feedback. | Leverages catalog to understand data sources and model capabilities for strategic planning. | Relies on observability to ensure product performance and user satisfaction. | Integrated insights for better strategic decisions and product development. |
13 | Business Analyst | 1. Conducting business-specific analysis.2. Reporting and visualization.3. Assisting in decision-making. | Helps in discovering relevant data assets and understanding the context behind the data. | They benefit from the assurance that the data they are using for reports is up-to-date and accurate. | Integrated tools mean faster access to reliable data, enhancing the speed and accuracy of business decisions. |
14 | Compliance Officer | 1. Regulatory compliance and reporting.2. Risk assessment.3. Policy enforcement. | Uses the data catalog for understanding data provenance and ensuring regulatory compliance. | Observability tools help in monitoring for breaches and non-compliance in real-time. | The convergence can streamline compliance reporting and risk assessment processes, making them more efficient. |
15 | AI Model Monitor | 1. Monitoring model performance post-deployment.2. Identifying and diagnosing model drift or unexpected behaviors.3. Implementing model updates and patches. | References the catalog to understand data lineage and the context of model decisions. | Leverages observability to detect and alert on model anomalies in real-time. | Enables quicker response to model issues through combined insights on data lineage and model performance. |
Wrapping Up
Data catalogs and data observability tools are like the nervous and immune systems of an organization’s data body. They work together to keep the data flowing to the right places, keep it healthy, and react quickly if something goes wrong. This coordination is critical to smooth and effective DataOps and MLOps processes, helping organizations to deploy and maintain data and ML-driven solutions with confidence and agility.
A comprehensive data catalog becomes indispensable as we navigate an era of rapidly expanding data and AI. It is a detailed map for organizations to understand and utilize their data resources effectively. However, the dynamic nature of data landscapes demands more than static mapping; data observability steps in as a real-time navigator, constantly updating and ensuring the accuracy and safety of these data pathways.
The fusion of data cataloging and observability creates a dynamic and trustworthy blueprint for data landscapes, transforming raw data into actionable insights and empowering informed decision-making. This integration is crucial for harnessing the present-day potential of data and sets the stage for future advancements. It paves the way for autonomous, self-correcting data ecosystems that can proactively manage discrepancies, streamline compliance, and unlock new growth opportunities. This convergence is key to shaping the future of data-driven innovation and possibilities.
About Author
Ankur Gupta brings a wealth of experience in product marketing, product management, and business analytics. He most recently served as the Product Marketing Director at Collibra, a data governance and catalog leader. Before that, Ankur held key positions at Talend, Reltio, Krux, and Yahoo.Ankur earned his MBA from Cornell University and engineering degrees from the Indian Institute of Technology Delhi. He currently resides in San Jose with his wife and two young children.
- On this page
See what’s possible with Telmai
Request a demo to see the full power of Telmai’s data observability tool for yourself.