Telmai Announces $2.8 Million Seed From .406 Partners, Zetta Venture Partners, Y Combinator & others
Telmai, a no-code real-time data quality analysis and monitoring platform, has successfully closed its Seed round with .406 Ventures, Zetta Venture Partners, Y Combinator, and notable angel investors. This milestone marks a significant step in Telmai’s journey, with plans for future growth and a commitment to a machine learning-first approach for proactive data quality monitoring.
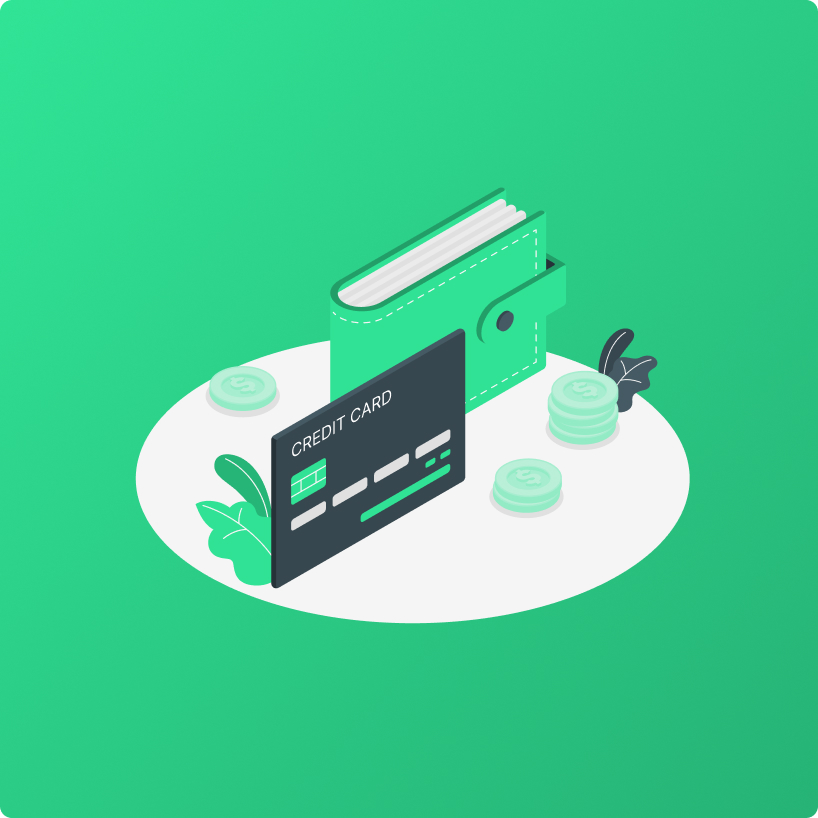
June 18, 2022
We have some exciting news to share – we’ve closed our Seed round with .406 Ventures, Zetta Venture Partners, Y Combinator, and some exceptional angel investors like, Manish Sood (Founder and CTO, Reltio), Sam Ramji (Chief Strategy Officer, DataStax) and Venkat Varadachary (CEO, Zenon | Former EVP/CDAO @ Amex).
We feel fortunate to partner with Graham Brooks and Jocelyn Goldfein on our journey. They are the best partners we could have asked for as a data company with an ML-first approach.
This is a big milestone for our team. We wanted to take this moment to share our thoughts on Telmai’s opportunity, and our plans for the future.
Telmai is a no-code real-time data quality analysis and monitoring platform. Our product automatically and proactively detects data quality issues as data is being ingested. Our human-in-the-loop machine learning engine enables Telmai users to define correct versus incorrect data -definitions that in turn enable proactive and autonomous monitoring and alerting of data quality issues.
Company momentum
Since inception in November 2020, we’ve been working hard to build visionary products and spread the word about our unique approach to data reliability. We have been fortunate to work with early design partners like Dun & Bradstreet and Myers-Holum, who share our vision for the future of data quality. Thanks to partners like them, and the thousands of prospective users we’ve been fortunate to interview, Telmai launched its MVP this month (October 2021).
More important than our category-leading product is our world-class team. We have been fortunate to recruit a lean but exceptional technical group who share a deep belief in Telmai’s vision of the future.
The next chapter
This seed funding round has enabled us to grow our team and to continue building Telmai’s leading data-quality platform for the modern data stack.
We are excited to continue this journey with an incredible group of institutional and angel investors who believe in our mission.
Investor Quotes
“As an investor focused on data and analytics for the past 15 years, our portfolio and our network of Chief Data Officers have continually highlighted data quality and trust as key challenges to realizing the promise of big data analytics and machine learning.
The biggest challenge around data quality is the historic gap between engineering-focused data quality tools and business users, who understand the data, how it is being used and the expectations around data reliability. Telmai is not only eliminating that gap but also addressing both IT and business’ most pressing needs in an infinitely-scalable, integrated data observability tool that establishes trust in data.”
– Graham Brooks, Partner, .406 Ventures
“The AI and machine learning platform shift is well under way, and ensuring data quality – efficiently, in production, and at scale – will be a prerequisite for every product and every business decision in the decades to come. We’re delighted to partner with Telm.ai’s founders, who acutely understand the challenge of maintaining high quality data and have built an industry leading product, itself powered by data and machine learning, to help their customers achieve it.”
– Jocelyn Goldfein, Managing Director , Zetta Venture Partners
We want to thank our customers and all the people who made it possible for us to reach this point – we are beyond excited to continue Telmai’s journey.
Onwards
– Mona & Max
- On this page
See what’s possible with Telmai
Request a demo to see the full power of Telmai’s data observability tool for yourself.
Articles
See all articles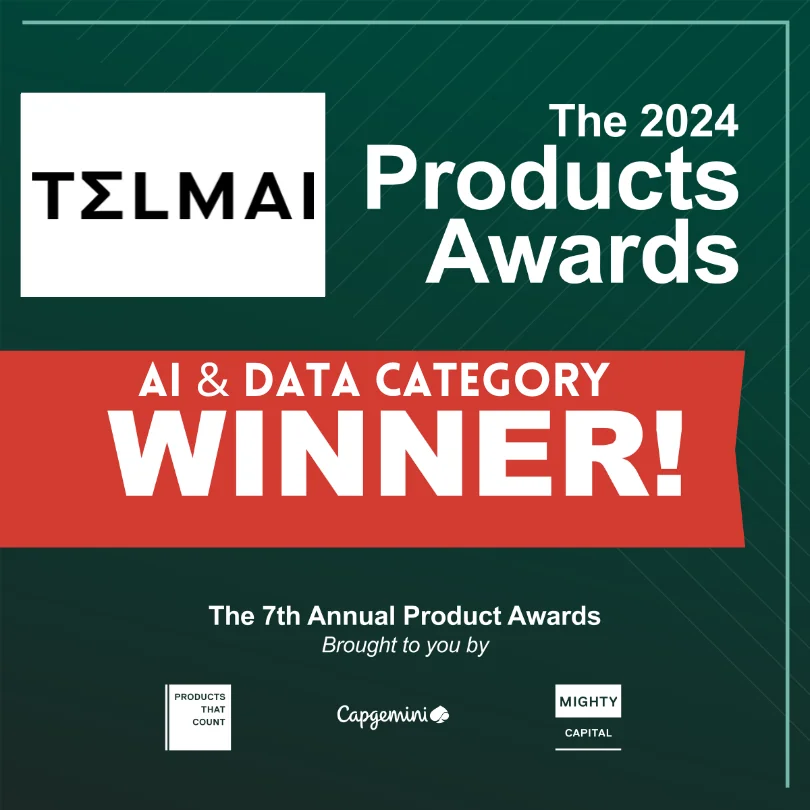
Telmai Awarded Best Product in AI & Data
Mar 14, 2024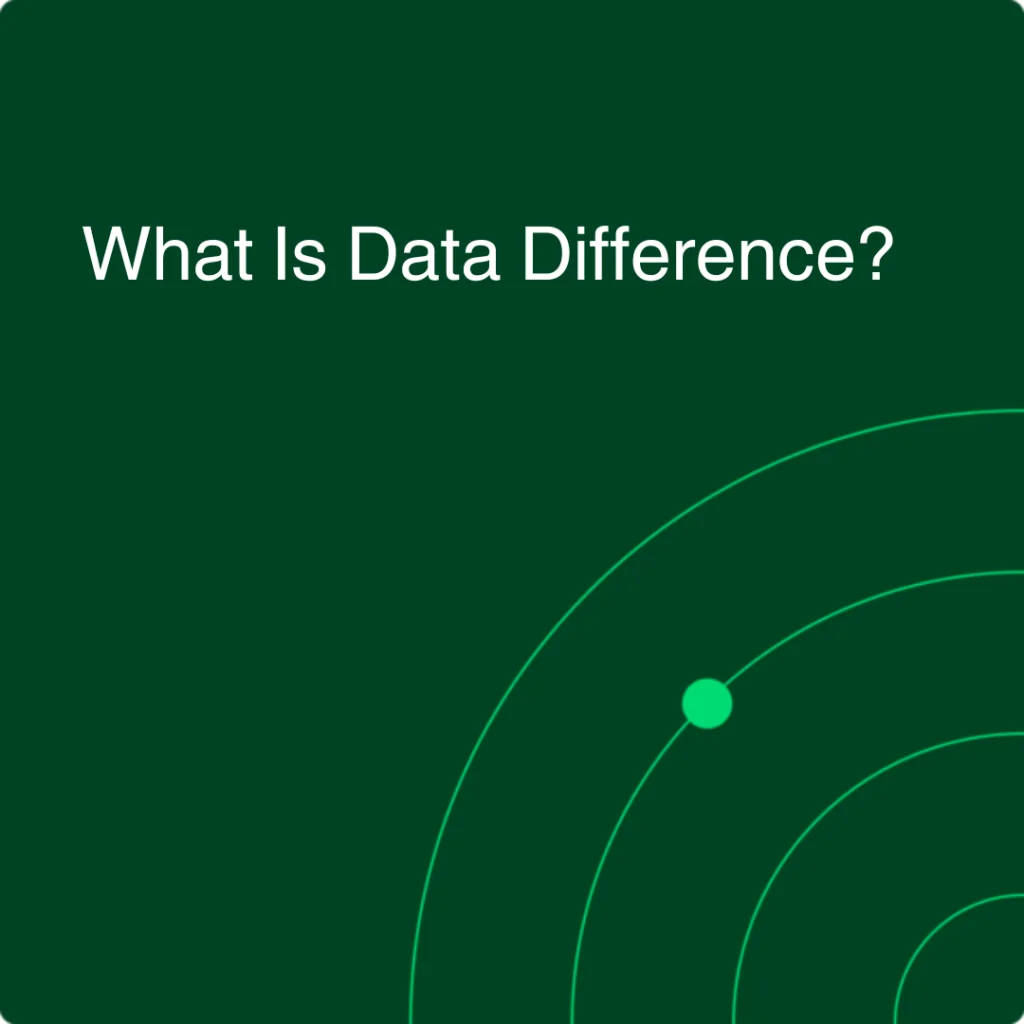
Data Difference: What Is It And Why Do You Need It?
Mar 08, 2024Telmai’s Integration For Alation
Jan 19, 2024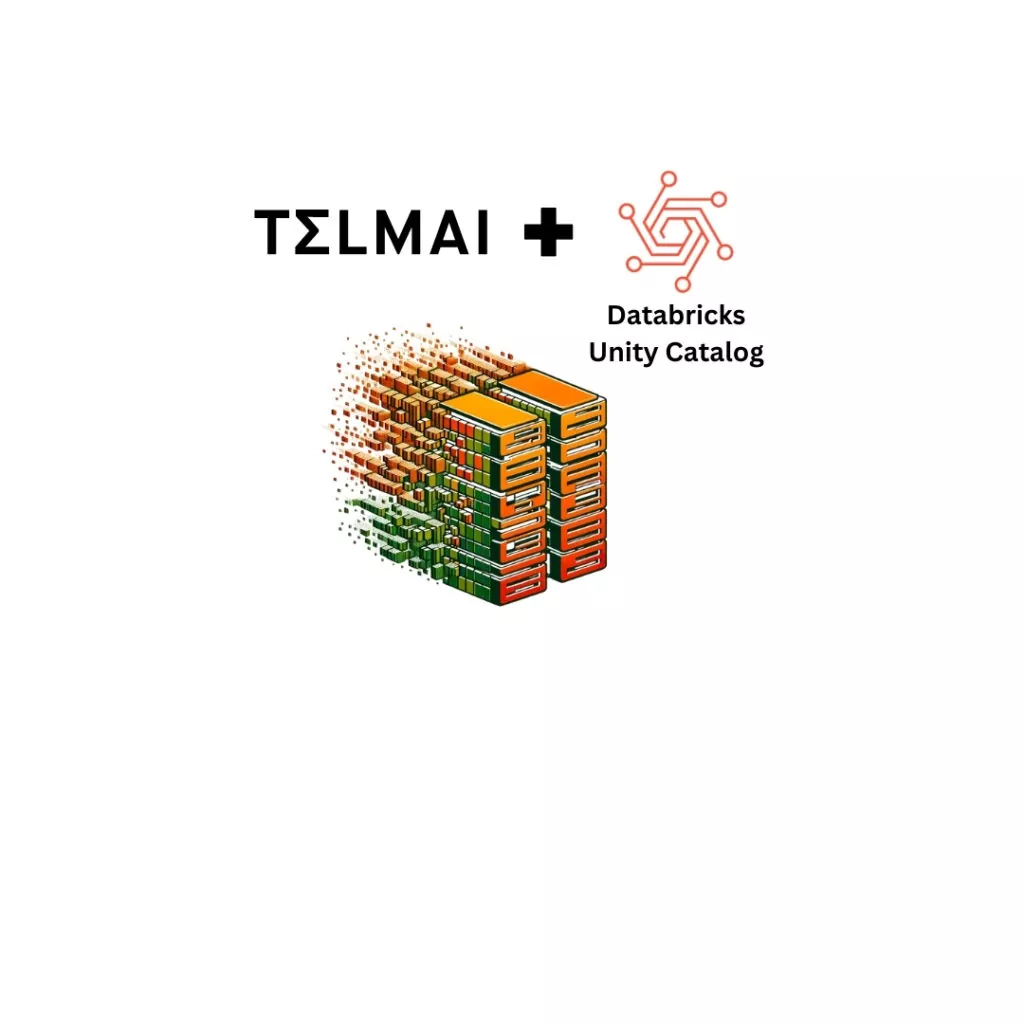