How to do data quality checks in SQL
Duplicates, null values, data types, and more. Delve into these sophisticated techniques and discover Telmai – a revolutionary tool that takes these concepts to the next level.
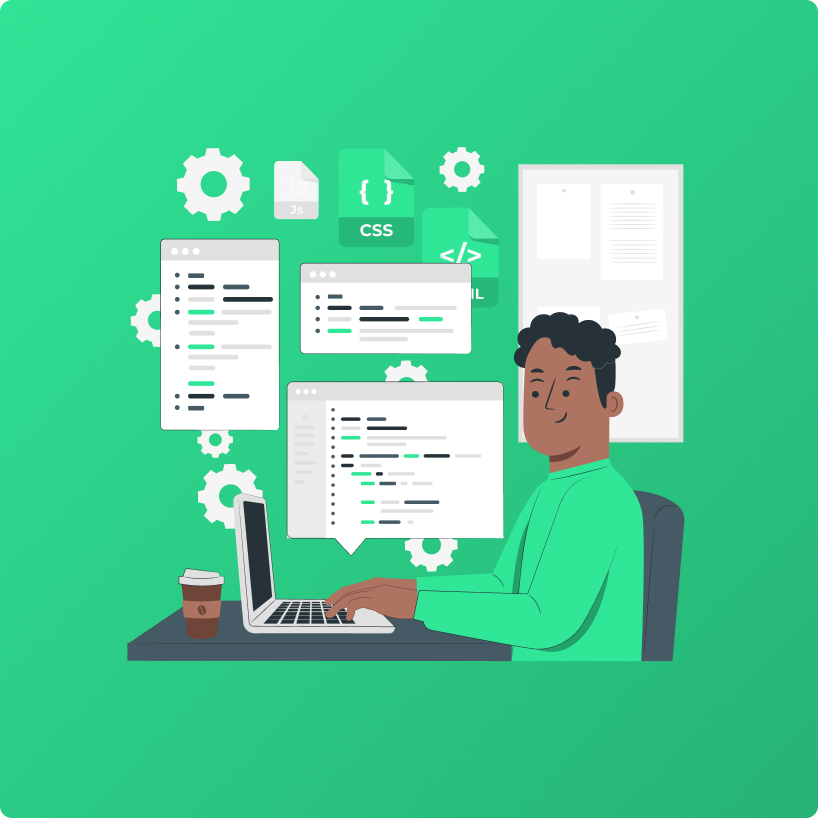
Did you know that you can do more with SQL than just retrieve and manipulate data?
By periodically querying your database to track critical metrics – such as row count, percentage of unique values in a column, and other basic data quality KPIs – you can check and maintain the health of your tables.
This post will cover 9 data quality checks you can perform with SQL. You will also learn about Telmai, a high-performance alternative to SQL-based tools that can automate data quality checks with a low-code, no-code interface, and without slowing down your databases.
Duplicate records check
First we’ll identify duplicate records in a table.
A duplicate records check in SQL can be performed by using the GROUP BY clause along with the HAVING clause. Here’s an example in SQL Server:
SELECT column_1, column_2, ..., column_n, COUNT(*)
FROM table_name
GROUP BY column_1, column_2, ..., column_n
HAVING COUNT(*) > 1;
In this code, the GROUP BY clause groups together records with identical values in the specified columns, and the HAVING clause filters out groups with a count of one, leaving only the duplicates.
NULL value check
Next, let’s ensure that mandatory fields have values rather than NULLs.
A null value check in SQL can be performed by using the IS NULL operator in a WHERE clause. Here’s an example:
SELECT
[column1], [column2], ..., [columnN]
FROM
WHERE [columnX] ISNULL;
This will give you a result set of all the records in the table where the value in column X is NULL.
Data type check
Now let’s verify that data in each field is of the correct data type.
A data type check in SQL can be performed by using the CASE statement and the IS OF operator. Here’s an example:
SELECT
[column1], [column2], ..., [columnN],
CASE
WHEN [columnX] IS OF (DATATYPE1) THEN 'DataType1'
WHEN [columnX] IS OF (DATATYPE2) THEN 'DataType2'
ELSE 'Other'
END AS DataType
FROM
[table_name];
This will return all the records in the table, along with a new column, DataType, indicating the data type of the value in columnX. The CASE statement checks the data type of columnX and returns a string value indicating the data type. In this example, DATATYPE1 and DATATYPE2 are placeholders for the actual data types you want to check for, such as INT, VARCHAR, DATE, etc. If the data type of columnX is neither DATATYPE1 nor DATATYPE2, it will be categorized as “Other.”
Range check
Here we validate that values in a field fall within a specified range.
A range check in SQL can be performed by using the BETWEEN operator in a WHERE clause. Here’s how:
SELECT
[column1], [column2], ..., [columnN]
FROM
WHERE [columnX] BETWEEN [lower_bound] AND [upper_bound];
This will give you all the records in the table where the value in columnX is between lower_bound and upper_bound. The BETWEEN operator is inclusive, meaning that the result set will include records where the value in columnX is equal to lower_bound or upper_bound.
Domain check
A domain check in SQL can be performed by using a subquery in a WHERE clause to check if the value in a column is in a list of valid values, known as a domain.
Here’s the example query:
SELECT
[column1], [column2], ..., [columnN]
FROM
WHERE [columnX] IN (SELECT [valid_value1], [valid_value2], ..., [valid_valueN] FROM [domain_table]);
This gives you all the records in the table where the value in columnX is in the list of valid values stored in the domain_table.
Uniqueness check
This helps to prevent duplicates from being inserted into the database and ensures that each value in the column is unique.
The most common way to perform a uniqueness check in SQL is to use a unique constraint. This is a restriction placed on a column or set of columns that ensures that the values in those columns are unique across all records in the table.
Here’s an example of how to create one:
ALTER TABLE [table_name]
ADD CONSTRAINT [uc_column_name]
UNIQUE ([column_name]);
This will create a unique constraint uc_column_name on the column_name in the table_name. If you try to insert a record into the table with a value that already exists in the column_name, the database engine will raise an error and reject the insertion.
Format check
A format check is a way to enforce a particular pattern or format for the values in a particular column, helping to ensure that the data in the column is consistent.
Here’s an example:
ALTER TABLE [table_name]
ADD CONSTRAINT [ck_column_name]
CHECK ([column_name] LIKE '[A-Z][0-9][0-9]');
This will create a check constraint ck_column_name on the column_name in the table_name. The constraint ensures that the value in column_name starts with an uppercase letter and is followed by two digits. Knowing regex will help you form the constraint.
Length check
A length check is a way to enforce a specific length or range of lengths for the values in a particular column.
Here’s an example using a check constraint:
ALTER TABLE [table_name]
ADD CONSTRAINT [ck_column_name]
CHECK (LEN([column_name]) >= 5 AND LEN([column_name]) <= 10);
This will create a check constraint ck_column_name on the column_name in the table_name. The constraint ensures that the length of the value in column_name is between 5 and 10 characters.
Completeness check
Last but not least, a completeness check helps to identify missing or incomplete data and prevent it from affecting your analysis or reports.
There are a few ways to perform a completeness check in SQL:
Use the NULL value
You can check for missing data by looking for NULL values in a particular column.
SELECT [column_name]
FROM [table_name]
WHERE [column_name] IS NULL;
This query returns all the rows where the column_name is NULL.
Use the COUNT function
You can also use the COUNT function to check the completeness of your data.
SELECT COUNT([column_name])
FROM [table_name];
This query returns the number of rows in the table_name. You can compare this count to the expected number of rows to determine if there is any missing data.
Use the GROUP BY clause
You can also use the GROUP BY clause to check for missing data.
SELECT [group_by_column], COUNT([column_name])
FROM [table_name]
GROUP BY [group_by_column];
This query returns the number of rows for each unique value in the group_by_column. You can use this information to determine if there is any missing data for a particular group.
Why SQL-based data quality checks aren’t enough
Developing a tool in-house sounds appealing since you know your domain and can easily define all the SQL queries you want to monitor your data. In addition, it seems relatively simple to implement an alerting application that runs queries against your databases and notifies you based on your defined rules.
However, an in-house tool comes with its own difficulties. The following are the five main drawbacks to this approach:
- They only work with data sources that can be queried in SQL. If you have to monitor streaming data, data coming from cloud file storage, flat files, APIs, or NoSQL databases, you’re out of luck. Limiting monitoring to only SQL sources often means that you are not able to monitor the data upstream of that SQL source.
- They will slow down your databases. Building a scalable SQL-based monitoring application is difficult because such applications work by executing queries at constant time intervals. As your database gets bigger, the entire monitoring process slows down due to the extra time needed to query hundreds or thousands of attributes and millions of records. You might instead choose to run queries more sparingly, but this can prevent you from finding out about problems until they’ve grown large enough to threaten your workflow.
- They add processing costs to your infrastructure. Your costs will go up if you need to execute a lot of queries on your database. To avoid performance problems, as discussed in the previous point, you need to increase the capacity of your infrastructure and dedicate more resources to it, which in turn increases your costs.
- They are hard to maintain over time. Data is inherently subject to change. As your data changes, you would need to change your data quality checks. This means having an inventory of all your rules along with proper documentation and downstream impacts they may have to be able to tweak and adjust them without implications.
- They miss the unknown unknowns. You can only validate or check what you know. That means anomalies, outliers, and drifts in your data may get passed the rules you set up without you knowing.
An alternative option
The real key to improving your data monitoring process is to begin monitoring before the data lands in your data warehouse. As an example of an alternative option, let’s take a look at what Telmai offers:
Telmai gives you the ability to monitor data across your entire pipeline regardless of whether your data structures are in SQL or nonSQL, so you can track and analyze your data upstream or as it’s being transferred to your database or data warehouse. This means you can monitor incoming data without impacting the performance of your data warehouse. You’ll also learn about potential problems with the data sooner in the process.
Telmai’s data quality analysis and observability platform is designed to help you detect and solve issues in real time, in every stage of your data transformation. Unlike using SQL to assess the health of data when data is at rest in the database or data warehouse, with Telmai, you won’t need to write any code or rules to set it up. Its user-friendly UI allows you to define what correct data should look like, and its machine learning algorithms proactively monitor the data and alert you to deviations that you may not have accounted in the past.
To see how Telmai can speed up and improve your data monitoring, request a demo.
Passionate about data quality? Get expert insights and guides delivered straight to your inbox – click here to subscribe to our newsletter now.
- On this page
See what’s possible with Telmai
Request a demo to see the full power of Telmai’s data observability tool for yourself.