Enhancing financial insights with data quality
Learn from this blog how prioritizing data quality in the financial services industry is essential for compliance, risk management, and decision-making, enhancing operations through real-time accuracy and advanced tools.
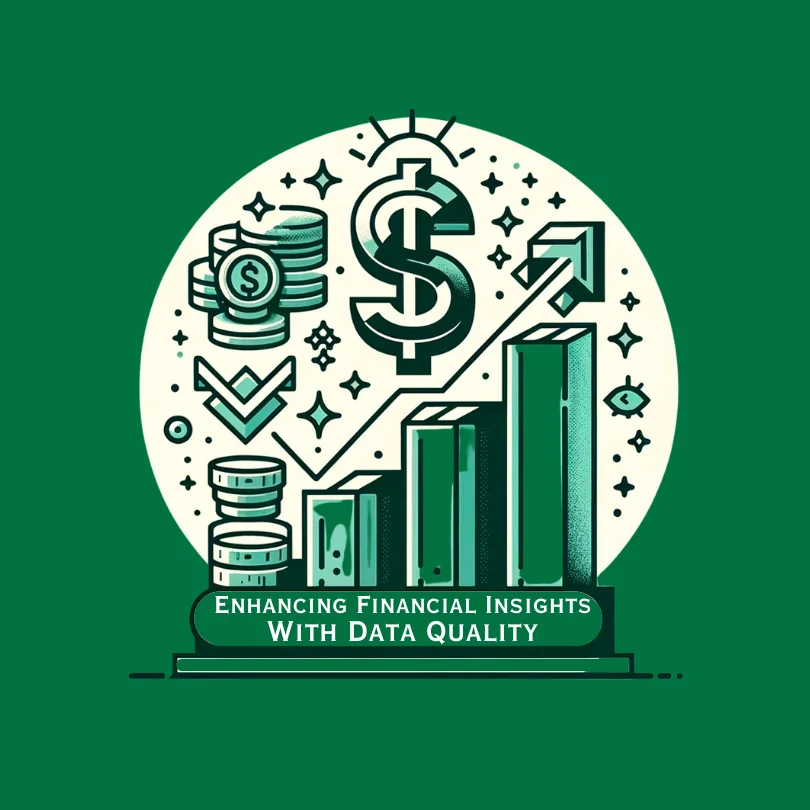
Financial services are tightly regulated sectors where compliance and risk management are critical. These institutions prioritize the continuous monitoring and reporting of data to regulatory bodies to maintain integrity and accuracy, given the massive volumes of data they handle. Even minor inaccuracies can significantly impact, leading to breaches of trust and severe repercussions. Poor data quality not only drains budgets silently through remediation efforts but also causes lost opportunities and inefficiencies. In the worst cases, a single error can drastically tarnish your reputation, resulting in regulatory fines and unwanted scrutiny.
Let’s begin by understanding the data quality in the financial sector and how data quality solutions can help tackle these related challenges.
Defining data quality in financial services
Data quality is crucial in shaping risk management strategies and ensuring regulatory compliance within the financial services industry. Data inaccuracies, such as incorrect counterparty identifiers or outdated Central Counterparty codes, can significantly jeopardize an organization’s reputation and economic stability. The necessity for immediate action in this sector means data errors can quickly propagate through operations. A data quality tool could swiftly identify such anomalies, minimizing remediation efforts and time.
A growing number of decision-makers require broader analytical contexts, supported by more granular financial and nonfinancial data, making data quality foundational for scalable decision-making in this sector, mainly through its adherence to accuracy, completeness, and timeliness standards, crucial for regulatory compliance.
Failure to maintain accurate data in critical operational areas can lead to severe consequences, including regulatory non-compliance, financial losses, and damage to stakeholder trust. For instance, incorrect counterparty identifiers could cause failed transactions or improper risk assessments, leading to potential legal and economic repercussions. Similarly, outdated Central Counterparty codes might disrupt clearing and settlement processes, causing delays and increasing operational costs. Therefore, implementing practical data quality tools and procedures is imperative to mitigate risks, ensure regulatory compliance, and safeguard the integrity of financial operations.
Moreover, data integrity holds critical significance in reporting, analytics, and forecasting in the financial domain. Data consistency can lead to better decision-making processes, heightened operational expenditures, and potential customer attrition. Implementing AI solutions in financial services also requires high-quality data, which serves as the foundational knowledge for training algorithms.
How can financial services boost data quality
Improving data integrity within the financial sector demands a multifaceted strategy. Data quality and Data Governance intersect notably in compliance, where ongoing data quality initiatives that focus on continuous monitoring bolster both domains. Embedding data quality initiatives within a robust data governance framework offers insights into data quality within the business’s operational context. This enables data stewards to pinpoint and triage data quality issues, allows business users to explore hypothetical scenarios, and facilitates data auditing for adherence to regulations like GDPR, CCAR, and other critical standards such as Basel III, FATCA, and EMIR.
Continuous data quality monitoring grants financial services firms enhanced oversight across their complete data pipelines, vital for operational and analytical functions. This data also provides the foundation for enabling AI applications to train machine learning models. Adopting a practical, self-service model ensures that data quality remains decentralized to all organizational stakeholders, empowering them to actively detect and address data quality challenges.
Scale your financial insights through data quality excellence
Employing automated data quality solutions guarantees data accuracy, thereby enhancing the efficiency of time-sensitive financial services and enabling the delivery of reliable results with less effort. Below are a few cases that highlight how it can be done.
- Mastering compliance, simplifying risk
Various fundamental financial operational workflows rely on standardized fields to accurately document transactional information, ensuring precision and compliance with regulatory requirements. These standardized fields cover diverse facets of economic activities, including but not limited to currencies, securities, commodities, and banking transactions. These fields are the backbone of financial systems, ensuring uniformity, accuracy, and interoperability across borders and markets.
Among these standardized fields, currency codes governed by the International Organization for Standardization (ISO) stand out as particularly vital, facilitating seamless transactions and providing essential clarity in the complex world of international finance.
The immense volume of data in the financial services industry renders manual validation and traditional methods impractical and error-prone. Missing or incorrectly formatted currency codes lead to inaccurate financial analyses, flawed risk assessments, regulatory non-compliance, and risk workflow breakdowns.
In response to these challenges, advanced data quality solutions have emerged, offering a proactive approach to mitigate risks and ensure accuracy in financial operations.
- Real-time insights, real-value gains
Financial organizations grapple with processing vast volumes of near-real-time data, presenting significant challenges in accurately tracking key metrics while ensuring proper correlations and eliminating duplicates. Furthermore, hidden patterns within data from reporting, exchanges, and source systems add complexity. Detecting improbable patterns before influencing data-driven decisions notably reduces the need for extensive remediation efforts.
Consider a scenario where anomalies emerge, such as when both the “Transaction Type” and “Transaction Status” fields are not null, yet their corresponding “Transaction Amount” value is either null or contains random, nonsensical values. This inconsistency could disrupt financial analyses, jeopardize risk assessments, and cause regulatory non-compliance.
Implementing comprehensive data quality solutions proves essential to tackle such challenges effectively. These solutions utilize sophisticated Machine Learning algorithms to detect deviations from historical patterns and continuously monitoring data streams for real-time outlier detection, ensuring the delivery of high-quality data pipelines to analytical models.
- Data consistency through simplified governance
The rapid adoption of cloud technology in the banking and financial sector marks a significant shift towards digital services over traditional face-to-face interactions. Cloud adoption promises enhanced agility and operational efficiency, underscoring the critical role of data governance and quality assurance.
Financial institutions frequently handle large volumes of data, necessitating seamless transfers between storage systems. However, this process poses inherent risks to data integrity, highlighting the importance of rigorous validation procedures to safeguard against alterations. Poorly managed and substandard data can hinder successful cloud migration, posing significant obstacles to overall financial operations’ efficiency.
Advanced data quality solutions, integrated with robust data governance frameworks, play a pivotal role in addressing these challenges. These solutions offer a suite of capabilities, including:
- Streamlining workflows for proactive resolution of data issues.
- Ensuring the integrity of data throughout migration processes using automatic profiling of sources
- Provide continuous, reliable data flow through your data pipelines by utilizing embedded data quality workflows like circuit breakers and Data Quality binning.
- Unlock operational efficiency with trusted data
Effectively managing credit risk is a top priority for financial institutions. Given the inherent uncertainties, meticulous data validation is indispensable throughout the loan underwriting and approval process to successfully mitigate credit risk. This validation encompasses critical fields such as credit scores, social security numbers (SSNs), loan-to-value ratios, and interest rates, and it prevents duplicate loan applications, ensuring the accuracy and integrity of loan-related information.
The adoption of decentralized data quality approaches holds significant potential in enhancing the management and validation of critical information. By seamlessly integrating business with other branches of the organization, these approaches streamline processes and minimize efforts in reconciliation and error management. This decentralized methodology guarantees the accuracy and integrity of data. It optimizes efficiency across essential departments, reinforcing overall operational effectiveness in bank lending.
Maximizing financial insights with Telmai
Leveraging data quality excellence is not just an advantage; it’s a necessity.
While there are plenty of other tools, Telmai sets itself apart by providing real-time, continuous data monitoring. It can detect anomalies and format inconsistencies while ensuring data remains fresh.
Telmai’s edge lies in its user-friendly interface and the deployment of AI-driven analytics, utilizing advanced machine learning algorithms to foresee and proactively address potential data quality issues. With its intuitive dashboard, users can effortlessly visualize data health, trace lineage, and understand quality metrics, empowering financial institutions to operate with the highest data integrity and analytical insight.
Discover how Telmai can revolutionize your data ecosystem, making it more dynamic and value-driven. Request a demo of Telmai today.
- On this page
See what’s possible with Telmai
Request a demo to see the full power of Telmai’s data observability tool for yourself.