How to Craft a Winning Data Quality Strategy
Treat each data issue as a product, prioritize fixes, design for users, and measure success with clear KPIs. Watch the full video below.
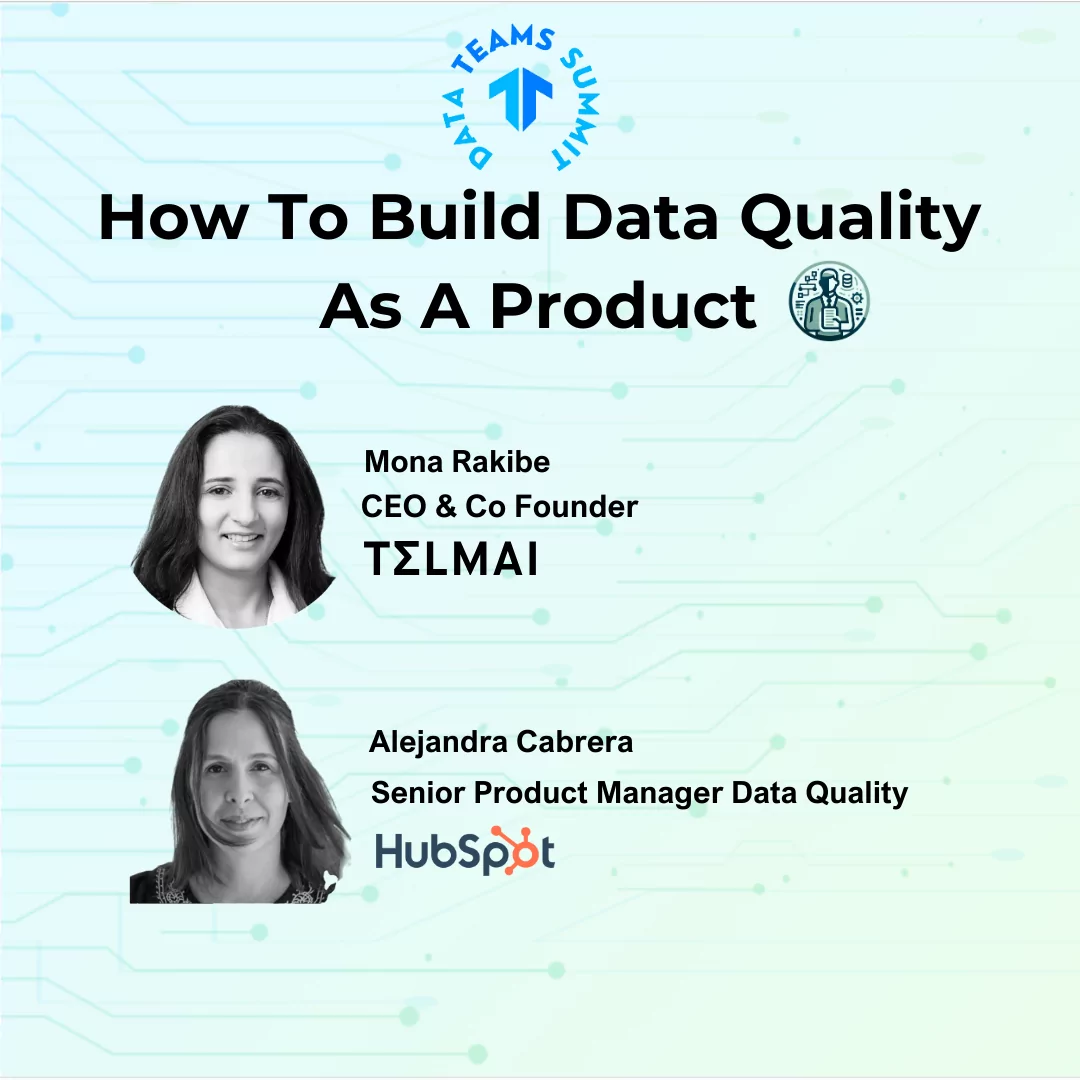
December 20, 2023
Two figures stood out at the recent Data Teams Summit: Alejandra Cabrera of Hubspot and Mona Rakibe of Telmai for their breakout session on How to build data quality as a product.
Alejandra and Mona propose treating data not as a byproduct of business but as the core product itself. It’s a subtle shift in perspective, but like the butterfly effect in chaos theory, it can potentially revolutionize outcomes.
In short, an effective data quality strategy means treating each data issue as a product, prioritizing fixes, designing for users, and measuring success with clear KPIs.
The journey from data as a raw input to a polished asset is fraught with challenges, but if you’re willing to embrace these practices, the rewards are as substantial as the datasets you manage.
1. Treat data quality as a product
This mindset shifts your approach from one of mere maintenance to one of creation and innovation. You need to start “adding more product thinking,” Cabrera advises.
This means adopting the product manager’s ethos, seeing beyond the immediate fix to architect a robust system that stands up to the demands of your users and the market.
Rakibe reinforces this approach: “Build a strategy for proper design, engineering, testing, and user adoption.” Your strategy should be as thorough and user-focused as if you were launching a new feature because, in essence, you are.
2. Define requirements and prioritize engineering work
This is about understanding what you need your data to do and the standards it must meet to serve its purpose effectively.
For instance, accuracy might mean less than 0.5% error rate in data entry fields, while timeliness could translate to data being refreshed every 24 hours.
Use a framework like the Eisenhower Matrix to categorize tasks by user impact, alignment with business goals, and resource availability, or adopt an Agile methodology to address issues based on their urgency and importance iteratively.
3. Build a strategy for proper design, engineering, testing, and user adoption
Here’s how you can approach this:
Design with the End User in Mind
Start by understanding the needs and pain points of those who will be interacting with your data. What do they require from the data to perform their jobs effectively? Design your data quality solution to solve these problems first. As Alejandra put it, “it’s about what is the product behind the scenes.” Your design should not only address the current issues but also anticipate future needs.
Engineer for Reliability and Scalability
Your engineering efforts should focus on building a robust system that can handle the volume and complexity of your data. This means choosing the right technologies and architectures to scale as your data grows. “We started the Telmai subscription, and we were able to start doing fixes,” shares Alejandra, highlighting the importance of selecting tools that enable you to continuously maintain and improve data quality.
Implement Rigorous Testing Protocols
Testing is critical. It’s not enough to assume your data quality initiatives are working; you must prove it through rigorous testing. Set up regular automated tests, checking for accuracy, completeness, and other quality metrics you’ve defined. This will help you catch issues before they affect users.
Facilitate User Adoption Through Training and Support
Finally, ensure that your users are prepared to adopt the new data quality measures. This might involve training sessions, detailed documentation, and ongoing support. As Rakibe suggests, “use product thinking.” Think about how users will interact with your data and what they need to know to get the most out of it. User adoption is the ultimate test of your data quality strategy’s success.
4. Create data quality health KPIs to measure the success of your work
To truly gauge the effectiveness of your data quality strategy, you must establish KPIs that reflect the health of your data. These KPIs should be carefully crafted to align with your business objectives and provide a clear measure of success.
Identify What Matters Most
Begin by pinpointing the aspects of data quality that are most critical to your business. Is it the accuracy of customer contact information, the completeness of product data, or the consistency of transaction records? For example, Clearbit might focus on the precision of data enrichment services, as it directly impacts customer satisfaction and retention.
Set Quantifiable Targets
Your KPIs need to be measurable. If accuracy is a priority, define what percentage of data accuracy is acceptable. If it’s completeness, determine what constitutes a complete record.
Regular Monitoring and Reporting
With your KPIs in place, establish a routine for monitoring and reporting. This could involve dashboards that provide real-time data quality metrics or regular audits to assess the state of your data. The goal is to create a feedback loop where data quality can be continuously assessed and improved upon.
Use KPIs to Drive Improvement
These metrics should not be static. As you collect data on your KPIs, use this information to drive your data quality initiatives. If certain targets are not being met, investigate why and determine what changes need to be made. This continuous improvement cycle is at the heart of a successful data quality strategy.
Communicate the Value
Finally, ensure that the significance of these KPIs is communicated across the organization. When stakeholders understand how data quality impacts the bottom line, they are more likely to support and engage with improvement efforts. It’s about showing how data quality translates into business quality.
Learn more about how Clearbit treats data quality as a product with Telmai
Telmai’s data observability platform scaled to analyze Clearbit’s data and provided the team with completeness, correctness, and volume metrics. “By October, we were able to have a dedicated monitoring…and the cool thing is by November, we were able to apply fixes and monitor the impact across the board,” said Cabrera.
Read Clearbit’s case study now.
Ready to elevate your data quality with ease? Request a demo of Telmai today.
- On this page
See what’s possible with Telmai
Request a demo to see the full power of Telmai’s data observability tool for yourself.
Articles
See all articles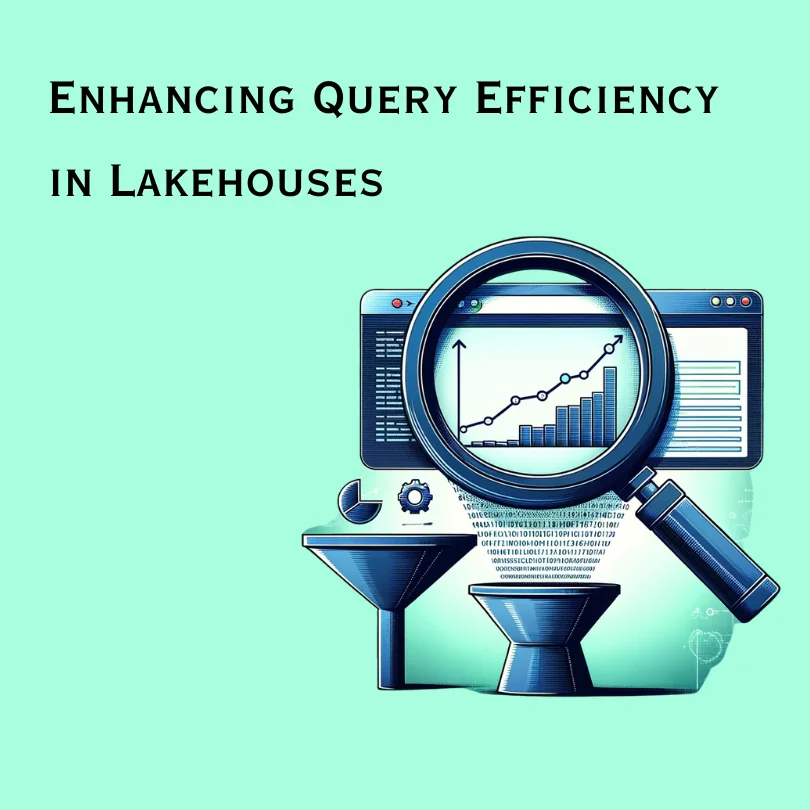