Who Is Responsible For Data Quality?
Collaboration and shared responsibility across business landscapes are key to unlocking the full potential of data-driven decision-making. Discover the transformative approaches reshaping how companies view and manage their most valuable asset: data.
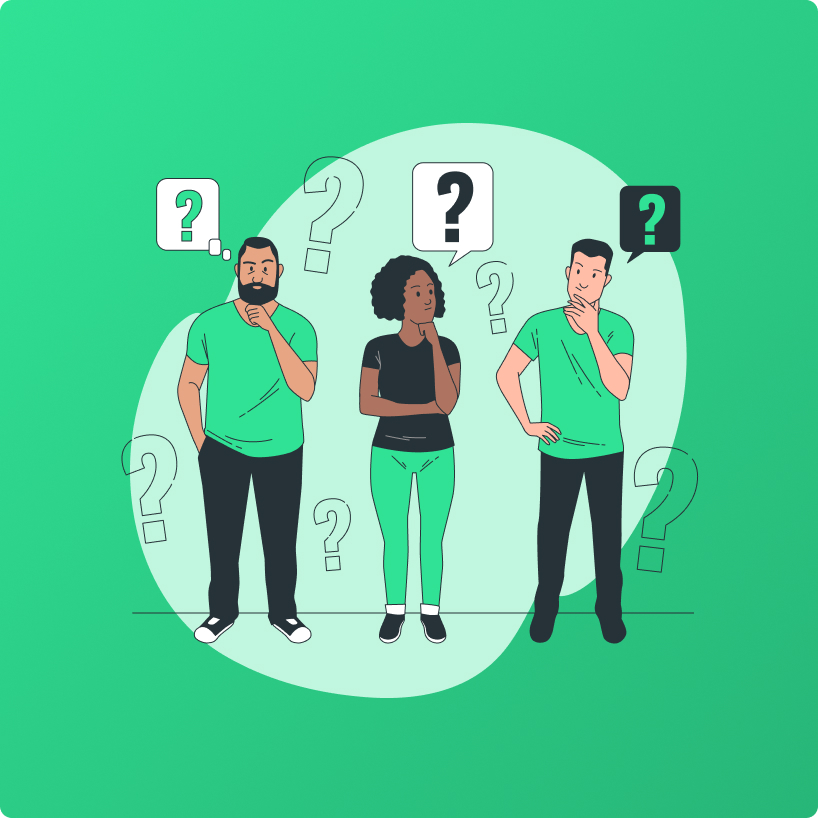
Responsibility for data quality depends on several factors: organizational structure, domain knowledge expertise, and the role of data in business enhancement.
According to 43% of respondents in our 2024 State of Data Quality Survey, data engineers are the most likely to own data quality. Only 14% had a separate data quality team.
Who is responsible for data quality?
Data quality ownership should be collaborative.
Adopting a culture around data means data quality ownership cannot be silo’d. Your people, tools, and processes should be structured around your sourcing, access, quality, and consumption needs. This requires bringing together both business owners and data engineers. Extending the core data ownership roles to business units allows for more domain-informed decision-making with the engineering team to support the larger vision.
So, how can such teams co-exist to form a solid data team?
There’s been no shortage of frameworks and architectural suggestions in the past few years trying to answer this question. Zhamak Dehghani, principal technology consultant at Thoughtworks, in her blog post How to Move Beyond a Monolithic Data Lake, breaks down models that data-driven organizations could consider to create a solid foundation for all data management. Two stand out in particular: the domain-oriented data ownership model and the domain-agnostic data ownership model.
Domain-Oriented Data Ownership Model
More traditional data architectures follow a linear, central, domain-oriented data ownership approach. A centralized data ownership team manages data ingestion from various sources, processes the data, and then provides access to different consumers, scientists, analytics, and BI teams.
This may prove to be a good solution for smaller organizations with simpler domain and consumption use cases, where a central team can serve the needs of a handful of distinct domains using data warehouse and data lake architectures. The key here is centralized data management, which is a hurdle for growth and expansive data vision. The data teams may be overwhelmed serving various needs and fighting fires to produce correct, timely data to make business-critical decisions.
Domain Agnostic Data Ownership Model
The centralized data ownership model will fail to keep up with a more complex infrastructure with many different sources of data and equally diverse sets of consumers with different needs and use cases. As Zhamak Dehghani emphasizes in this article, the necessity of transitioning to a data mesh reflects a fundamental shift in enterprise data platform strategies for handling such complexities.
While data pipeline, ingestion, and storage can be maintained by a centralized self-serve data infrastructure platform team, allowing data to be locally owned by product domains for their specific use case can allow for a more independent and targeted lifecycle of data, dictating quality measures, metadata structures, consumer needs, defining business KPIs for their product data thereby reducing the turnaround time in response to their customers and the overall load on the otherwise centralized data team.
Such teams would continue to include product data owners and data engineers to support the needs of the product and bring together a team of unique skills that fulfills the wider data-driven vision of the organization.
Democratize Data Quality Ownership
Business and data analysts are more accustomed to identifying issues with data in the context of its use case than the engineering teams. However, as described above, both are essential to data ownership. While the operations teams have varied consumption and domain-centered quality needs, the domain data teams can define the accuracy of the Service Level Indicators (SLIs) on data.
Here is a high-level breakdown of the needs of various operational units in a typical organization:
Unit | Roles & Responsibility |
MarketingOps | Owns the marketing systems, sole owner of the tools, tech, and data in those tools |
Sales and Revenue | Owns the customer and accounts data in CRM systems |
CRM admin/ops | Owns the CRM systems, sole owner of the tools, tech, and data in those tools. Can procure 3rd-party data for enrichment. |
Data Quality and Governance | Owner of entire company data. |
Data and Analytics Head | Owns the data that’s needed for business teams, cares about data in the business context, and uses data from different teams. |
Data Engineers | Owner of certain systems like data warehouses and overall pipeline. Will check trends on data and pipeline health and often needs input from data stewards and analysts to understand good versus bad data, especially in large organizations. |
It’s very evident from our conversations that data quality can not be achieved by technology alone. It needs the right combination of process, culture, and tools, especially tools that will empower technical and non-technical teams.
To summarize,
Data quality needs to be democratized across business and IT, and this can be achieved when organizations focus on building a collaborative data culture.
More and more technologies need to focus on empowering entire data teams (both technical and non-technical) to improve data quality.
- On this page
See what’s possible with Telmai
Request a demo to see the full power of Telmai’s data observability tool for yourself.