Data quality: significance and consequences
Investing in a robust data management architecture is crucial for organizations to get a good ROI on data spends, with the quality of data playing a significant role in a data-driven organization. Identifying data anomalies at the foundation of the pipeline ensures accurate downstream data consumption, boosting business significantly.
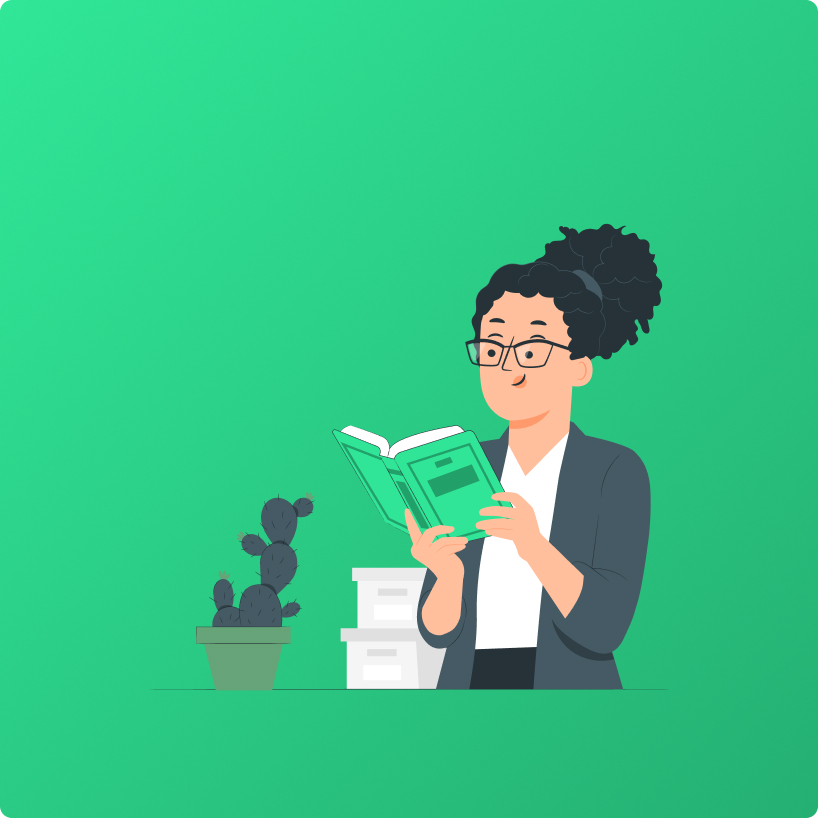
Significance and Consequence of data quality
Most organizations are recognizing the importance of investing in a robust and scalable data management architecture to get good ROI on data spends. Whether it is via DataOps or Data Mesh, you need to identify your unique selling point by placing trust in the most important asset – DATA.
Whether you collate data, or buy it, you know your output is only as good as the confidence you have in the input – Garbage In, Garbage Out. The quality of data is quite significant in a data-driven organization, and to identify data anomalies at the foundation of the pipeline step, will ensure all downstream consumption of data will accurately provide the results that can significantly boost your business.
How do we define data quality? Simply put, is the data accurate, in the right format, reliable and consistent. Just by analyzing the condition of data on some parameters can isolate the problem areas, thus forcing you to evaluate at the origin rather than at the end of the data lifecycle. There are many ways of identifying data anomalies, thereby improving data quality, such as data monitoring and observability, and we’ve described that in great detail in our post.
3 reasons why quality plays a big role in a data-driven organization
Here, I would like to stress on 3 of the many reasons why quality plays a big role in a data-driven organization.
- Making sound decisions: If the data being consumed by various different organizational processes is clean, and valid, the output will then help in making critical decisions that prove to be sound and have reduced risk. For example:
- Improved customer experience and targeted marketing: With data that is correct and timely, you can interact and provide the best service to your customers with the information you have on file. How often are they reading the newsletters, what kind of material is driving them to click vs. glance through, are customers responding to your ads are just some of the important pieces of information that can help you drive more targeted marketing channels.
- Productive Data Engineering Team: Research finds that most data engineers are fighting fires with issues in data, writing static rule based scripts to catch anomalies which soon become outdated or need to be supported continuously, taking away time from other core data engineering roles. By diverting attention from identifying leaks in data to high-yielding work, the ROI is much significant.
Sure, good data does have a great impact, but it’s often times taken for granted, because the opposite is more obvious – untrustworthy data can have tremendous consequences –
- Lost competitive advantage: With bad data, many profitable opportunities are simply overlooked or missed. Are you catering to the market and customers, with the right services and products that have an immediate buy-in? Are you speaking to the right audience, at the right time? If you’re not gaining insights by making good use of your assets, the competition is already ahead of the game.
- Decisions based on incorrect data affects reputation and can tend to create mistrust. Sectors with strict regulations, sanctions, trade need to be over cautious about mis-steps, sharing wrong information, overlooking fraudulent activities, or reaching out to the wrong customer base due to incorrect data. As recently as last week, a man was offered the COVID vaccine due to incorrect height and BMI calculation. Most likely, the data team hadn’t even anticipated the downstream use of such data to calculate BMI and plan vaccinations. It’s more evident than before that Data Quality checks should not be done only at the level of few attributes but anomaly detection should happen for most attributes in your data set.
- Revenue loss: The bottom line for most businesses is to effectively use all the resources on hand to increase revenue. According to a Gartner research, “organizations believe poor data quality to be responsible for an average of $15 million per year in losses.” All the reasons above and many more directly impact the revenue. For instance, due to ineffective marketing, when a sales channel fails to convert, the revenue is directly impacted.
Telm.ai can help identify anomalies and inaccuracies in your data, saving you time, effort and money. It seamlessly injects into your pipeline step, becoming an integral part of your data architecture.
#dataquality #dataanomalydetection #dataobservability #datamonitoring
About the Author
Harsha Bipin, Technical Marketing @ Telm.ai, Software Engineer and a big proponent of mindful living 🙂
Passionate about data quality? Get expert insights and guides delivered straight to your inbox – click here to subscribe to our newsletter now.
- On this page
See what’s possible with Telmai
Request a demo to see the full power of Telmai’s data observability tool for yourself.