Data Observability vs. Data Quality
Understand their definitions, differences, and how they contribute to effective data management. A must-read for anyone seeking to maximize the value of their data.
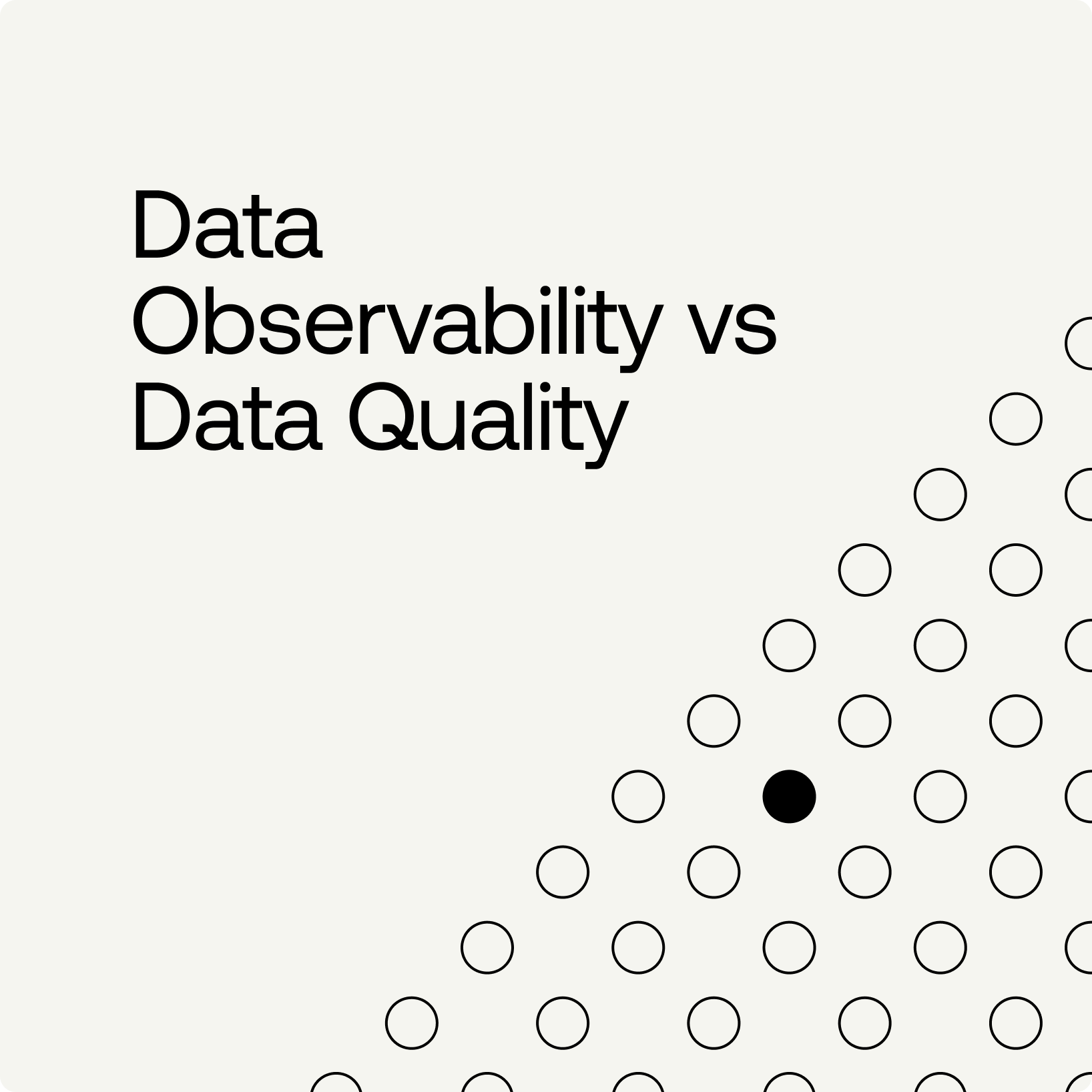
January 11, 2023
Data quality and data observability are two important concepts in data management, but they are often misunderstood or confused with one another. Understand the what, why, and how of each and you’ll be better equipped to get the most value out of your data.
First, let’s define both terms. Data quality is the state of data. It answers the question, “is the data usable and relevant?” Often this is identified using indicators like accuracy, completeness, freshness, correctness, and consistency.
Data observability, on the other hand, is a set of techniques that answers the question, “does the data contain any signals that need investigating?” By nature, data observability is continuous and provides real-time or near real-time insights about the data.
As the state of data changes, data observability is able to observe, capture, and notify us about the change in data. This observation could be about data quality issues or about signals in data that although considered healthy from a data quality standpoint, are significant nonetheless. Anomalies, outliers, or drifts in business data such as an unexpected change in a transaction amount fall into this category.
What can be done with data quality and data observability
Data quality focuses on validating data against a known set of policies. This provides a consistent understanding of the health of the data against predefined metrics.
Data observability, on the other hand, leverages ML and statistical analysis to learn from data and its historic trends and identify potential issues not previously known, and predict data changes.
Often the learnings from data observability can also be classified into data quality KPIs, hence accelerating data quality by automating the outcomes of data observability.
Data observability can also further investigate these issues and find root causes, therefore shortening the time to remediate data quality issues.
Upon finding issues and drifts in the data, data observability enables orchestrating and operationalizing data workflows. For example, with data observability, data teams can automate the decisions around the next steps of the pipeline.
Why data observability and data quality are essential to maintaining trust in your data
Data quality assesses the health of the data against predefined rules and expectations. For example, the uniqueness of social security numbers, or valid zip codes within a region. This validation helps data teams look for known or expected issues in order to create analytics or prepare data for data models.
Data quality requires a team – typically technical – to maintain, tweak and test data quality rules continuously.
Data observability, alternatively, detects drifts and anomalies that are outside the realms of data quality and could be unknown. It is a smarter system that helps automate identifying and alerting on both predicted (known) and unexpected (unknown) data changes.
A well-designed data observability tool is equipped with ML and automation to enable business teams to also participate in data quality and data monitoring initiatives.
Unlike traditional data quality tools, data observability tools are low-code / no-code with faster time to value and low cost of implementation and management.
How to implement data observability and data quality
Any organization that depends on data for key business decisions needs:
- Visibility into the latest health of the data via data quality KPIs
- Proactive and automated insights into any new and unexpected issues to handle them before any business impact
Any data team should be equipped with the tools to address these requirements.
Traditional Data quality tools
These tools focus on the validation, cleansing, and standardization of data to ensure its accuracy, completeness, and reliability.
Writing validation rules in SQL or using open source tools is one way to implement data quality. Traditional ETL tools often have data quality rules embedded in their user interface to transform the data into higher quality.
Traditional data quality tools work best for structured data. In order to analyze the health of semi-structured data or data that is in motion and streaming, further programming is needed to transform the data into an analytic-ready format.
To report on data quality issues and historical trends, the output of data validation checks needs to be built into a BI and visualized.
Data observability tools
While data observability tools can also validate data against predefined metrics from a known set of policies, they leverage ML and statistical analysis to learn about data and predict future thresholds and data drifts.
Given their constant monitoring and self-tuning nature, data observability tools can automatically curate issues and signals in the data into data quality KPIs and dashboards to showcase the ongoing data quality trends visually. These tools are equipped with interactive visualizations to enable investigation and further analysis.
Alerts and notifications are often table stakes and do not require programmatic configuration.
More sophisticated data observability tools are also capable of handling data stored in a semi-structured format or streaming data. These well-integrated platforms can span and serve complex data pipelines, in ways that data quality tools can not.
Use a tool like Telmai to orchestrate and operationalize your data workflows and automate the decisions around the next steps of the pipeline.
- On this page
See what’s possible with Telmai
Request a demo to see the full power of Telmai’s data observability tool for yourself.
Articles
See all articles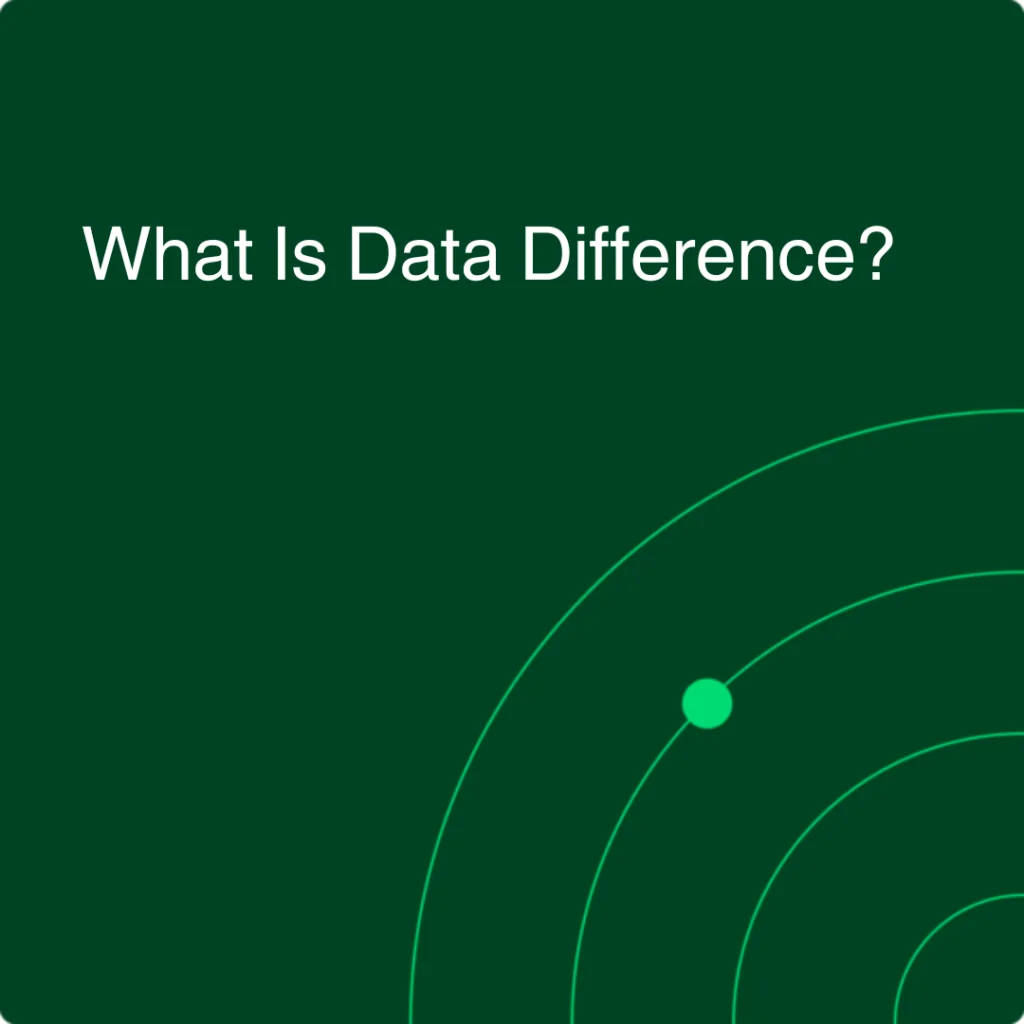
Data Difference: What Is It And Why Do You Need It?
Mar 08, 2024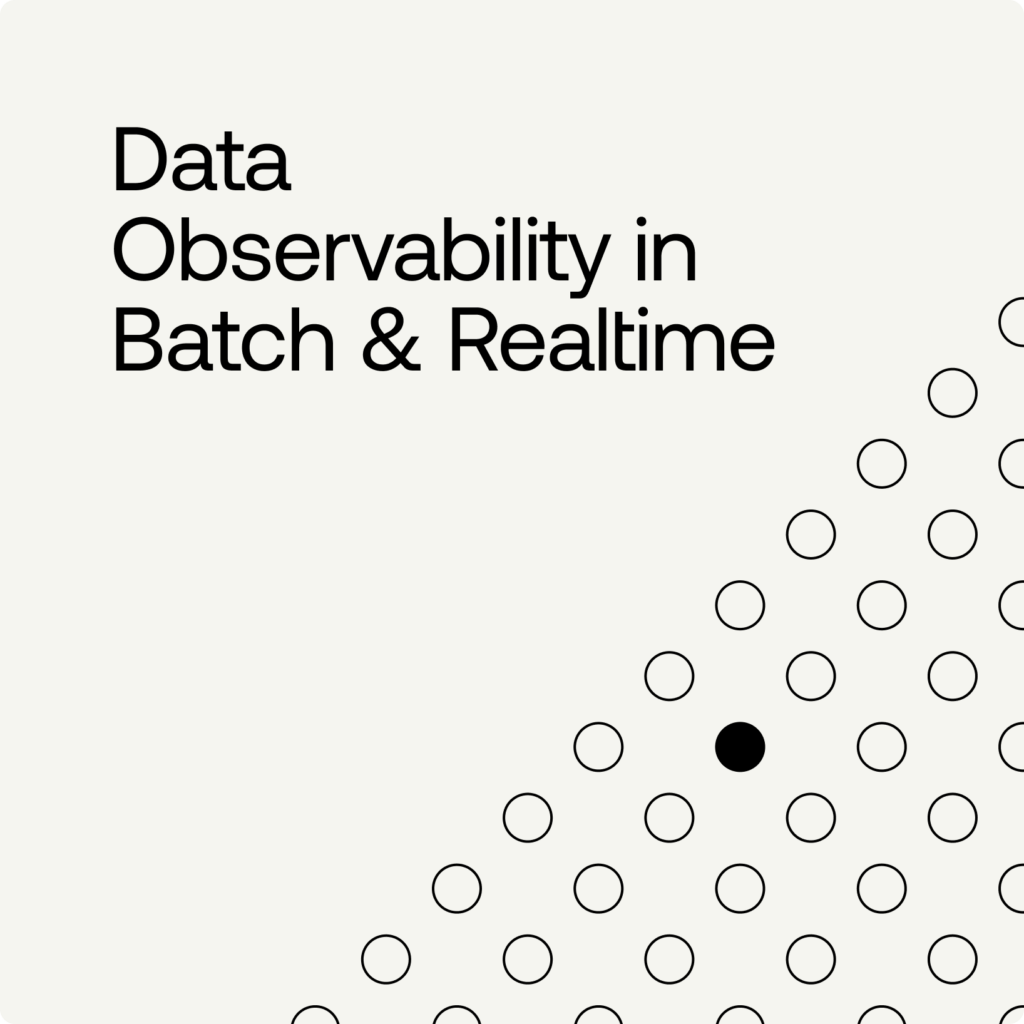
Data Observability in Batch and Realtime
Jun 25, 2023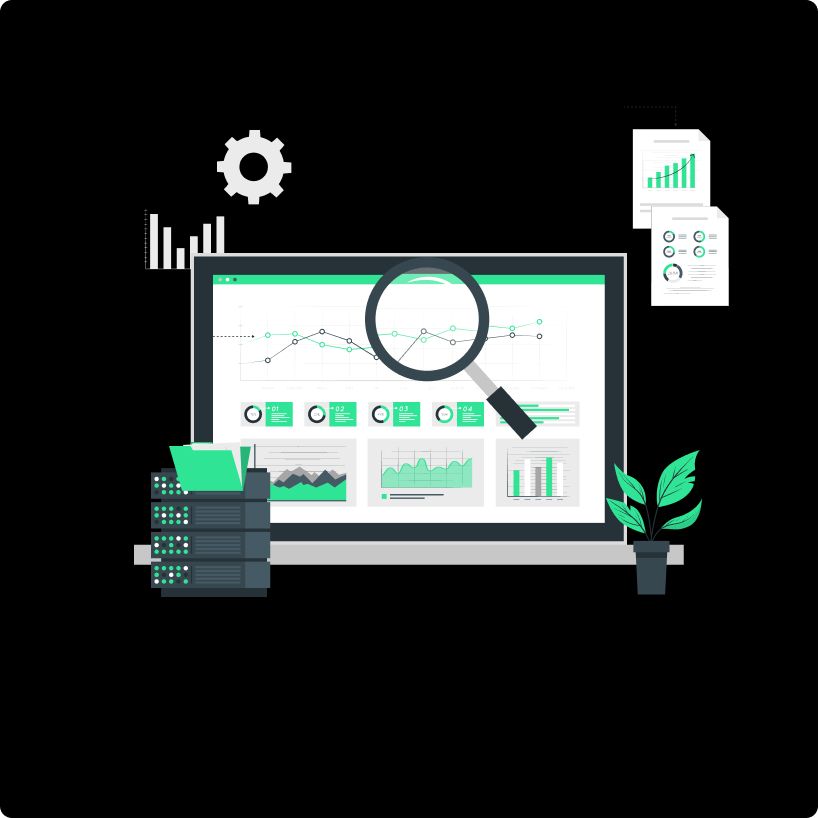