Data observability vs. metadata observability: which one do you need?
Dive into the differences between Data Observability and Metadata Observability. Understand their unique roles in data management, how they provide insights into data state, shape, form, value, and changes over time. Learn why both are crucial, yet offer different solutions to the same problem.
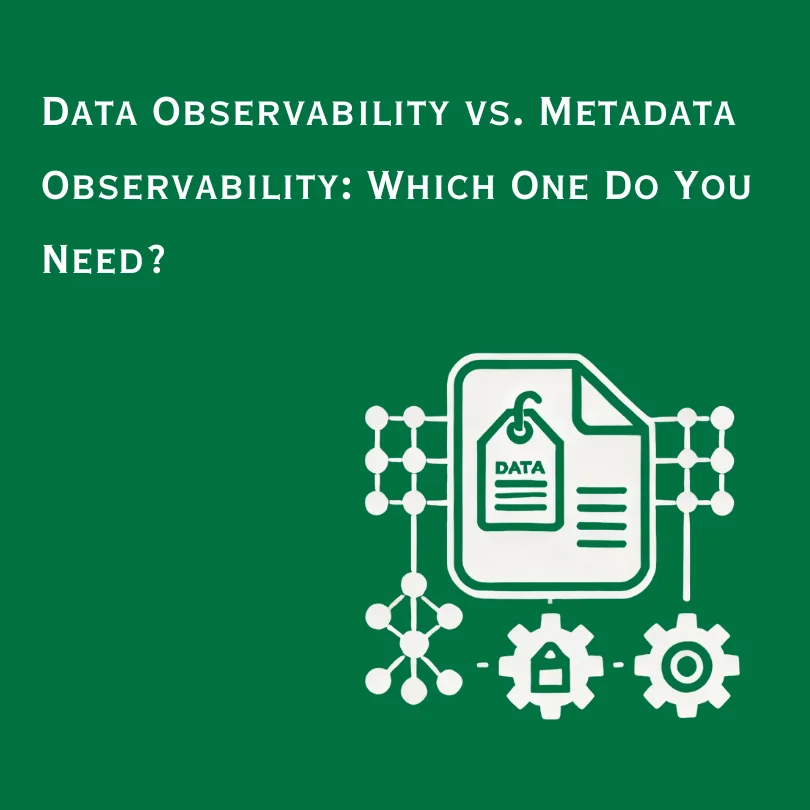
Introduction
What do you think the two shapes above have in common?
Would you have guessed that they have the same perimeter? Well – they do!
The perimeter of these shapes are the same, but they are not the same shape; they don’t have the same dimensions, their angles are not the same size, and their colors are different. In fact, one of them has a very small dot embedded inside it, not even noticeable at first glance.
This is exactly what Data Observability and Metadata Observability have in common and also not so in common.
What is data observability?
Data Observability is the degree of visibility you have in the data at any given point. Data Observability is all about understanding and monitoring the behavior, quality, and performance of your data as it moves through a system. Think of it as having a real-time, detailed map of your data’s journey, allowing you to keep an eye on its reliability, accuracy, and compliance.
Several key aspects come into play in achieving effective data observability:
- Data Quality: Accuracy, completeness, and consistency.
- Data Flow: Movement through systems and identification of bottlenecks.
- Data Dependencies: Relationships and impacts of changes.
- Data Anomalies: Detection of outliers and errors.
- Data Compliance: Adherence to regulations and policies
What is metadata observability?
Metadata – being data about data – doesn’t exactly know what is inside. It only infers information about the data, given limited facts about it. Any SQL database readily provides this information about its tables and federated views. For example, the number of rows in a table, the last it was updated, the range or min/max values in its various columns, its primary key, and whether the table saw some schema change, such as columns that were dropped or added.
Metadata Observability in the analogy above only gives us the perimeter of the data.
Comparing data and metadata observability
Let’s look at an example. Would a table that was updated in the last hour indicate that its data is fresh and reliable? What is the barometer to determine freshness? Is it only a timestamp? Maybe, maybe not. What if I tell you that just a few rows were updated and not the whole table? What if the table was updated, but it collected some garbage? Is the data in the table fresh, and is it reliable?
While Metadata Observability looks at data about the data, Data Observability, on the other hand, looks at the actual data itself and its values. It is able to validate the accuracy of the data. It can identify that the data has drifted during an update and that the number of anomalies has increased or decreased.
Additionally, metadata can not be the observability gauge for complex datasets such as semi-structured sources, streaming data, data directly coming from an application, or data retrieved by APIs. These data sources do not conform to a data model and often lack proper metadata.
To better illustrate the differences between data observability and metadata observability, here’s a table that provides a clear comparison:
Data Observability | Metadata Observability |
---|---|
When you need to monitor all your data across every stage of the pipeline. | When you only want to monitor your data warehouse and other structured databases. |
When a peripheral view of data recency and volume levels gives you peace of mind. | When you can rely on only metadata updates to see schema changes in your operational data stores. |
When you need a granular look at your data’s actual values, enabling you to spot anomalies and drifts directly within the data. | When you want to concentrate on high-level information regarding operational data stores, such as schema changes or row counts, providing an overview rather than deep insights. |
Conclusion
Understanding the differences between data observability and metadata observability helps in choosing the right tool for your needs. Integrating both can provide a balanced approach, offering both high-level oversight and detailed insights. The right data observability platform can help you maintain robust data management and quality, ensuring that your data is always reliable and actionable.
For further reading, dive into our comprehensive guide, “4 Types of Data Observability – Which One is Right for You.” This guide breaks down the various types of data observability solutions, their pros and cons, and helps you identify the best fit for your specific data needs and strategy.
Passionate about data quality? Get expert insights and guides delivered straight to your inbox – click here to subscribe to our newsletter now.
- On this page
See what’s possible with Telmai
Request a demo to see the full power of Telmai’s data observability tool for yourself.